Celest Sorrentino, MSc student, OSU Dept of Fisheries, Wildlife and Conservation Sciences, GEMM Lab
The first memory I have of A.I. (Artificial Intelligence) stems from one of my favorite movies growing up: I, Robot (2004). Shifting focus from the sci-fi thriller plot, the distinguished notion of a machine integrating into normal everyday life to perform human tasks, such as converse and automate labor, sparks intrigue. In 2014, my own realization of sci-fi fantasy turned reality initiated with the advertisements of self-driving cars by TESLA. But how does one go from a standard tool, like a vehicle, to an automated machine?
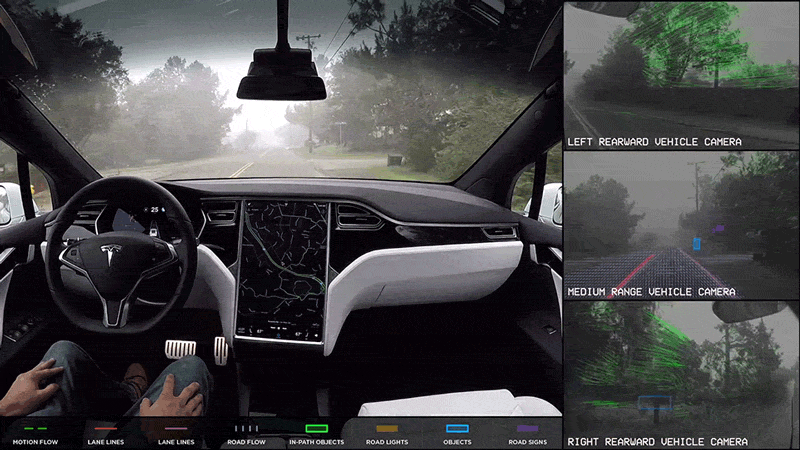
For my first thesis chapter, I am applying a machine learning model to our lab’s drone video dataset to understand whale mother-calf associations, which is in continuation of my previous internship in 2022. A.I. has absolutely skyrocketed in marine science and hundreds of papers have confirmed the advantage in using machine learning models, such as in species abundance estimates (Boulent et al 2023), whale morphometrics (Bierlich et al 2024), and even animal tracking (Periera et al 2022). Specifically, Dr. KC Bierlich recently led a publication on an incredible A.I. model that can extract still images from drone footage to be subsequently used for body morphometric analysis. Earlier this year my lab mate Nat wrote an insightful blog introducing the history of A.I. and how she uses A.I. for image segmentation to quantify mysid swarms. For those of us who study animal behavior and utilize video-based tools for observation, A.I. is a sweet treat we’ve been craving to speed up and improve our analyses —but where do we start?
With a Venn Diagram and definitions of course!
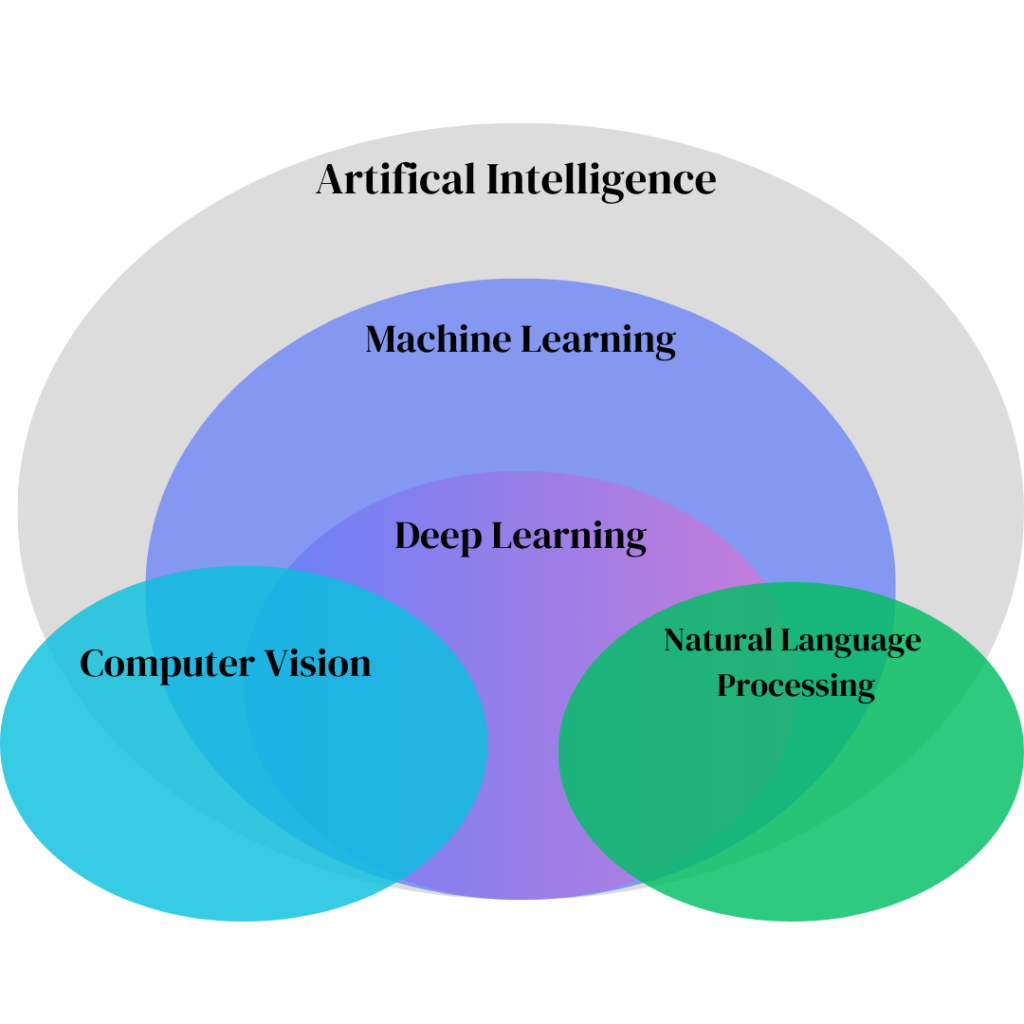
Good terms to know:
Artificial Intelligence: a machine/model built to mimic human intelligence.
Machine Learning: a subset of A.I. that uses statistical algorithms to recognize patterns and form predictions, usually requiring human intervention for correction.
Deep-learning: a specific form of machine learning that is meant to mimic human neural networks through artificial neural networks (ANN) by recognizing hierarchal patterns with minimal to no human-intervention to correct.
Computer Vision: a type of machine learning that enables a machine/model to gather and retain information from images, video, etc.
Natural Language Processing: a subset of machine learning in which a machine/model to identify, understand, and create text and speech.
(Still a bit confused? A great example of the difference between machine learning and deep-learning can be found here)
So, you have a dataset, what’s the pipeline?
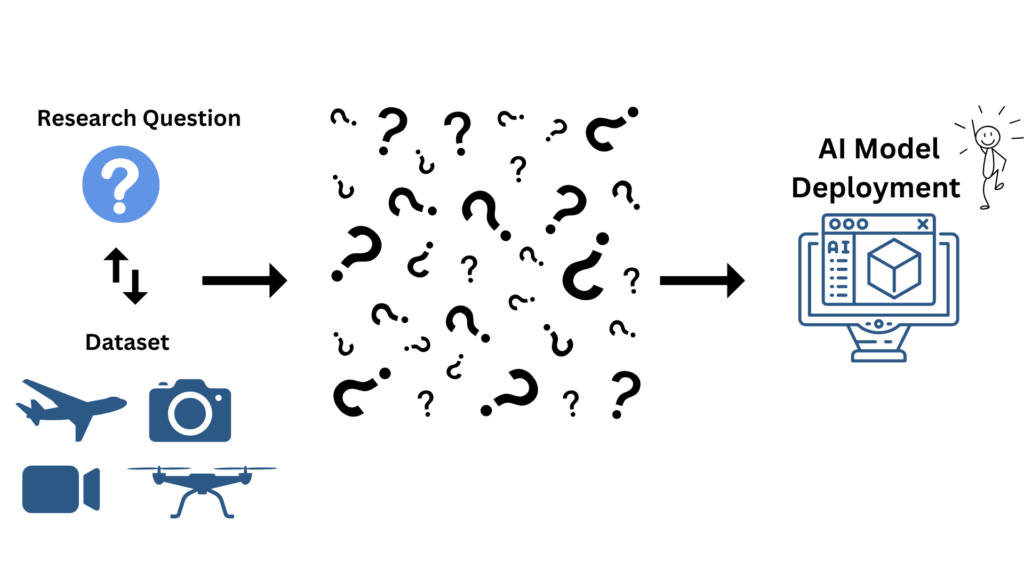
First, we must consider what type of data we have and our question. In fact, you might find these two questions are complimentary: What type of questions does our dataset inspire and/or what type of dataset is needed to answer our question?
Responses to these questions can guide whether A.I. is beneficial to invest in and which type to pursue. In my case, we have an imagery dataset (i.e., drone videos) and our question explores the relationship of mom-calf proximity as an indicator of calf-independence. Therefore, a model that employs Computer Vision is a sensible decision because we need a model that extracts information from imagery. From that decision, I then selected SLEAP A.I. as the deep-learning model I’ll use to identify and track animals in video (Pereira et al 2022).
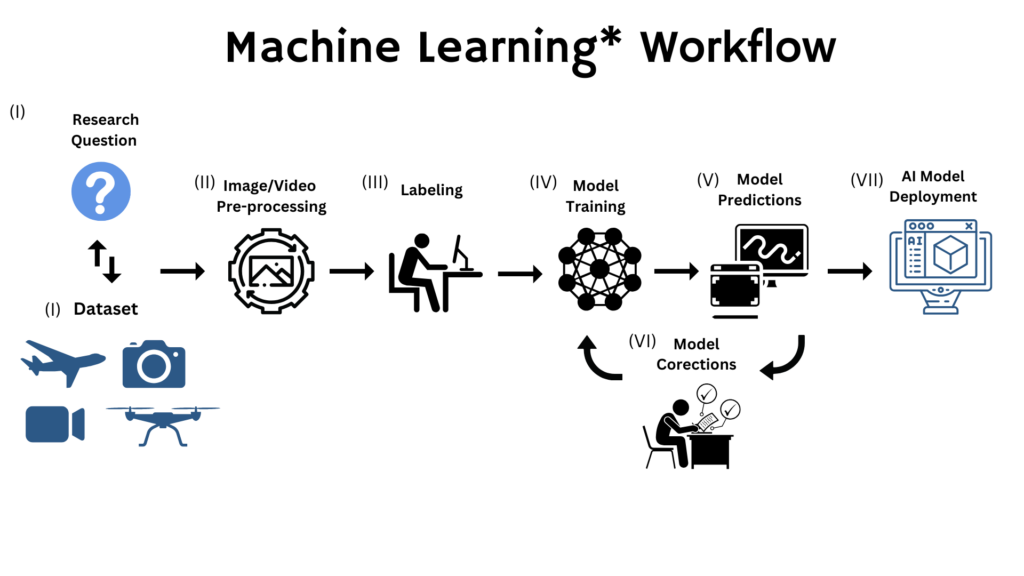
Why is image pre-processing important?
Image pre-processing is an essential step in “cleaning” the imagery data into a functional and insightful format for the machine learning model to extract information. Although tedious to some, I find this to be an exciting yet challenging step to push my ability to reframe my own perspective into another, a trait I believe all researchers share.
A few methods for image/video preprocessing include Resizing, Grayscaling, Noise Reduction, Normalization, Binarization, and Contrast enhancement. I found the following definitions and Python code by Maahi Patel to be incredibly concise and helpful. (Medium.com)
• Resizing: Resizing images to a uniform size is important for machine learning algorithms to function properly. We can use OpenCV’s resize() method to resize images.
• Grayscaling: Converting color images to grayscale can simplify your image data and reduce computational needs for some algorithms. The cvtColor() method can be used to convert RGB to grayscale.
• Noise reduction: Smoothing, blurring, and filtering techniques can be applied to remove unwanted noise from images. The GaussianBlur () and medianBlur () methods are commonly used for this.
• Normalization: Normalization adjusts the intensity values of pixels to a desired range, often between 0 to 1. This step can improve the performance of machine learning models. Normalize () from scikit-image can be used for this.
• Binarization: Binarization converts grayscale images to black and white by thresholding. The threshold () method is used to binarize images in OpenCV.
• Contrast enhancement: The contrast of images can be adjusted using histogram equalization. The equalizeHist () method enhances the contrast of images.
When deciding which between these techniques is best to apply to a dataset, it can be useful to think ahead about how you ultimately intend to deploy this model.
Image/Video Pre-Processing Re-framing
Notice the deliberate selection of the word “mimic” in the above definition for A.I. Living in an expeditiously tech-hungry world, losing sight of A.I. as a mimicry of human intelligence, not a replica, is inevitable. However, our own human intelligence derives from years of experience and exposure, constantly evolving – a machine learning model** does not have this same basis. As a child, we began with phonetics, which lead to simple words, subsequently achieving strings of sentences to ultimately formulate conversations. In a sense, you might consider these steps as “training” as we had more exposure to “data.” Therefore, when approaching image-preprocessing for the initial training dataset for an A.I. model, it’s integral to recognize the image from the lens of a computer, not as a human researcher. With each image, reminding ourselves: What is and isn’t necessary in this image? What is extra “noise”? Do all the features within this image contribute to getting closer to my question?
Model Workflow: What’s Next?
Now that we have our question, model, and “cleaned” dataset, the next few steps are: (II) Image/Video Processing, (III) Labeling, (IV) Model Training, (V) Model Predictions, and (VI) Model Corrections, which leads us to the ultimate step of (VII) A.I. Model Deployment. Labeling is the act of annotating images/videos with classifications the annotator (me or you) deems important for the model to recognize. Next, Model Training, Model Predictions, and Model Corrections can be considered an integrated part of the workflow broken down into steps. Model Training takes place once all labeling is complete, which begins the process for the model to perform the task assigned (i.e., object detection, image segmentation, pose estimation, etc.). After training, we provide the model with new data to test its performance, entering the stage of Model Predictions. Once Predictions have been made, the annotator reviews these attempts and corrects any misidentifications or mistakes, resulting in another round of Model Training. Finally, once satisfied with the model’s Performance, Model Deployment begins, which integrates the model into a “real-world” application.
In the ceaselessly advancing field of A.I., sometimes it can feel like the learning never ends. However, I encourage you to welcome the uncharted territory with a curious mind. Just like with any field of science, errors can happen but, with the right amount of persistence, so can success. I hope this blog has helped as a step forward toward understanding A.I. as an asset and how you can utilize it too!
**granted you are using a machine learning model that is not a foundation model. A foundation model is one that has been pre-trained on a large diverse dataset that one can use as a basis (or foundation) to perform specialized tasks. (i.e. Open A.I. ChatGPT).
References:
Bierlich, K. C., Karki, S., Bird, C. N., Fern, A., & Torres, L. G. (2024). Automated body length and body condition measurements of whales from drone videos for rapid assessment of population health. Marine Mammal Science, 40(4). https://doi.org/10.1111/mms.13137
Boulent, J., Charry, B., Kennedy, M. M., Tissier, E., Fan, R., Marcoux, M., Watt, C. A., & Gagné-Turcotte, A. (2023). Scaling whale monitoring using deep learning: A human-in-the-loop solution for analyzing aerial datasets. Frontiers in Marine Science, 10. https://doi.org/10.3389/fmars.2023.1099479
Deep Learning vs Machine Learning: The Ultimate Battle. (2022, May 2). https://www.turing.com/kb/ultimate-battle-between-deep-learning-and-machine-learning
Jain, P. (2024, November 28). Breakdown: Simplify AI, ML, NLP, deep learning, Computer vision. Medium. https://medium.com/@jainpalak9509/breakdown-simplify-ai-ml-nlp-deep-learning-computer-vision-c76cd982f1e4
Pereira, T.D., Tabris, N., Matsliah, A. et al. SLEAP: A deep learning system for multi-animal pose tracking. Nat Methods 19, 486–495 (2022). https://doi.org/10.1038/s41592-022-01426-1
Patel, M. (2023, October 23). The Complete Guide to Image Preprocessing Techniques in Python. Medium. https://medium.com/@maahip1304/the-complete-guide-to-image-preprocessing-techniques-in-python-dca30804550c
Team, I. D. and A. (2024, November 25). AI vs. Machine learning vs. Deep learning vs. Neural networks. IBM/Think. https://www.ibm.com/think/topics/ai-vs-machine-learning-vs-deep-learning-vs-neural-networks
The Tesla Advantage: 1.3 Billion Miles of Data. (2016). Bloomberg.Com. https://www.bloomberg.com/news/articles/2016-12-20/the-tesla-advantage-1-3-billion-miles-of-data?embedded-checkout=true
Wolfewicz, A. (2024, September 30). Deep Learning vs. Machine Learning – What’s The Difference? https://levity.ai/blog/difference-machine-learning-deep-learning