By: Kate Colson, MSc Student, University of British Columbia, Institute for the Oceans and Fisheries, Marine Mammal Research Unit
One of the most frequent questions graduate students get asked (besides when you are going to graduate) is what their plans are after university. For me, the answer has always adamantly been continuing to do research, most likely as a government researcher because I don’t want teaching commitments to take away from my ability to conduct research.
However, one of the most fulfilling parts of my degree at University of British Columbia has actually been teaching four terms of a 100-level undergraduate science course focused on developing first-year students’ critical thinking, data interpretation, and science communication skills. My role in the course has been facilitating active learning activities that exercise these skills and reviewing material the students go over in their pre-class work. Through this course, I have experienced the teaching styles of six different professors and practiced my own teaching. As with any skill, there is always room for improvement, so when I had a chance to read a book titled How Learning Works: Seven Research-Based Principles for Smart Teaching (Ambrose et al. 2010), I took it as an opportunity to further refine my teaching and explore why some practices are more effective than others.
In the book, Ambrose et al. present principles of learning, the research surrounding these principles and examples for incorporating them into a university level course. Some of the principles gave me ideas for strategies to incorporate into my teaching to benefit my students. These described how prior knowledge impacts student learning and how to use goal-oriented practice and give feedback relative to target criteria that the students can apply to the next practice task. For example, I learned to be more conscious about how I explain and clarify course material to make connections with what the students have learned previously, so they can draw on that prior knowledge. Other principles presented by Ambrose et al. were more complex and offered a chance for greater reflection.
Beyond presenting strategies for improving teaching, the book also presented research that supported what I had learned firsthand through teaching. These principles related to the factors that motivate students to learn and why the course climate matters for learning. I have seen how student motivation is impacted by the classroom climate and culture put forth by the teaching team. Perhaps the most frustrating experiences I have had teaching were when one member of the teaching team does not see the importance of fostering a supportive course environment.
For this reason, my favorite assignments have been the Thrive Contract and the Group Contract. Each term, the Thrive Contract is the first major class activity, and the Group Contract is the first group assignment. These assignments serve as a means for everyone to co-create guidelines and expectations and establish a positive classroom culture for the rest of the term. After an exceptionally poor classroom experience my first time teaching, I have highlighted the importance of the Thrive Contract in all subsequent terms. Now, I realize the significance I lent this assignment is supported by the research on the importance for a supportive environment to maximize student motivation and encourage classroom engagement (Figure 1).
Another powerful lesson I have learned through teaching is the importance of clarifying the purpose of an activity to the students. Highlighting a task’s objective is also supported by research to ensure that students ascribe value to the assigned work, increasing their motivation (Figure 1). In my teaching, I have noticed a trend of lower student participation and poorer performance on assignments when a professor does not emphasize the importance of the task. Reviewing the research that shows the value of a supportive course climate has further strengthened my belief in the importance of ensuring that students understand why their teaching team assigns each activity.
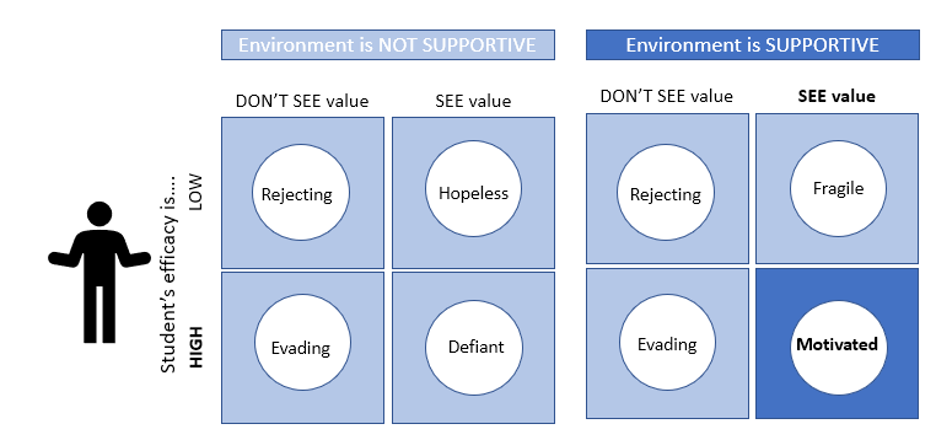
Potentially my favorite part about the structure of Ambrose’s book was that it offered me a chance to reflect not only on teaching, but also on my own learning and cognitive growth since I started my master’s degree. Graduate students are often in a unique position in which we are both students and teachers depending on the context of our surroundings. The ability to zoom out and realize how far I have come in not only teaching others, but also in teaching myself, has been humbling. My reflection on my own learning and growth has been driven by learning about how organizing knowledge affects learning, how mastery is developed and how students become self-directed learners.
One of the main differences between novices and experts in how they organize their knowledge is the depth of that knowledge and the connections made between different pieces of information. Research has shown that experts hold more connections between concepts, which allows for faster and easier retrieval of information that translates into ease in applying skills to different tasks (Bradshaw & Anderson, 1982; Reder & Anderson, 1980; Smith, Adams, & Schorr, 1978). Currently in my degree, I am experiencing this ease when it comes to coding my analysis and connecting my research to the broader implications for the field. By making these deeper connections across various contexts, I am building a more complex knowledge structure, thus progressing towards holding a more expert organization of knowledge.
In the stages of mastery concept proposed by Sprague and Stewart (2000), learners progress from unconscious incompetence where the student doesn’t know what they don’t know, to conscious incompetence where they have become aware of what they need to know (Figure 2). This was where I was when I started my master’s — I knew what objectives I wanted to achieve with my research, but I needed to learn the skills necessary for me to be able to collect the data and analyze it to answer my research questions. The next stage of mastery is conscious competence, in which the ability of the learner to function in their domain has greatly increased, but practicing the necessary skills still requires deliberate thinking and conscious actions (Figure 2). This is the level I feel I have progressed to — I am much more comfortable performing the necessary tasks related to my research and talking about how my work fills existing knowledge gaps in the field. However, it still helps to talk out my proposed plans with true masters in the field. The final stage of mastery, unconscious competence, is where the learner has reached a point where they can practice the skills of their field automatically and instinctively such that they are no longer aware of how they enact their knowledge (Figure 2).

In line with my progression to higher levels of mastery has come the development of metacognitive skills that have helped me become a better self-directed learner. Metacognition is the process of learning how to learn, requiring the learner to monitor and control their learning through various processes (Figure 3). The most exciting part of my metacognitive growth I have noticed is the greater independence I have in my learning. I am much better at assessing what is needed to complete specific tasks and planning my particular approach to successfully achieve that goal (e.g., the construction of a Hidden Markov model from my last blog). By becoming more aware of my own strengths and weaknesses as a learner, I am better able to prepare and give myself the support needed for completing certain tasks (e.g., reaching out to experts to help with my model construction as I knew this was an area of weakness for me). By becoming more aware of how I am monitoring and controlling my learning, I know I am setting myself up for success as a lifelong learner.
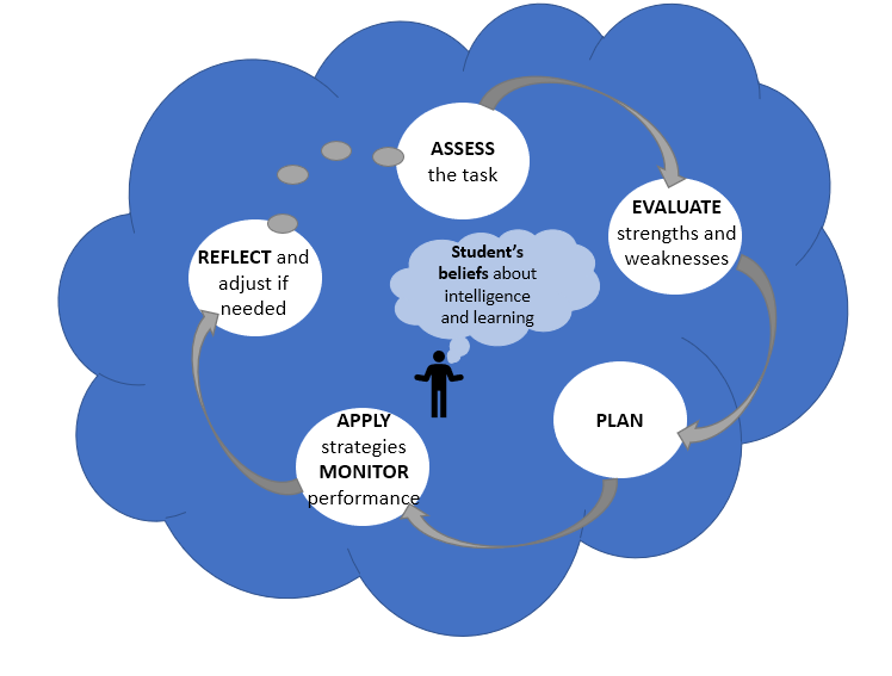
References:
Ambrose, S. A., Bridges, M. W., DiPietro, M., Lovett, M. C., & Norman, M. K. (2010). How learning works: Seven research-based principles for smart teaching (1st ed.). San Francisco, CA: Jossey-Bass.
Bradshaw, G. L., & Anderson, J. R. (1982). Elaborative encoding as an explanation of levels of processing. Journal of Verbal Learning and Verbal behaviours, 21,165-174.
Ford, M. E. (1992). Motivating humans: Goals, emotions and personal agency beliefs. Newbury Park, CA: Sage Publications, Inc.
Hansen, D. (1989). Lesson evading and dissembling: Ego strategies in the classroom. American Journal of Education, 97, 184-208.
Reder, L. M., & Anderson, J. R. (1980). A partial resolution of the paradox of interference: The role of integrating knowledge. Cognitive Psychology, 12, 447-472.
Smith, E. E., Adams, N., & Schorr, D. (1978). Fact retrieval and the paradox of interference. Cognitive Psychology, 10, 438-464.
Sprague, J., & Stewart, D. (2000). The speaker’s handbook. Fort Worth, TX: Harcourt College Publishers.
Zimmerman, B. J. (2001). Theories of self-regulated learning and academic achievement: An overview and analysis. In B. J. Zimmerman & D. H. Schunk (Eds.), Self-regulated learning and academic achievement (2nd ed., pp. 1-38). Hillsdale, NJ: Erlbaum.