Background
“In the Winter Term 2024, the Ecampus Research Unit conducted a survey study of 669 students who had taken online courses at OSU. The 40-item survey was designed to assess students’ knowledge and use of generative AI tools, as well as their perceptions of their use in their courses and careers. A full report of this study is available on the Ecampus Research Unit website. Based on the results of this study, several recommendations were developed to guide decision making about generative AI tools in online courses.”
Dello Stritto, Underhill, & Aguiar (2024).
This recent study highlighted three key recommendations for faculty seeking to integrate generative AI into their courses effectively:
- Recommendation 1
- Write a course policy about generative AI that is clearly explained.
- Recommendation 2
- Consider a wide range of student emotions and concerns when integrating generative AI in your online courses.
- Recommendation 3
- Educate students on generative AI tools.
Applying data to design
To apply these recommendations in practice, we can reorganize them into instructional design categories that foster AI resiliency in course design: Course Learning Outcomes, Learner Profiles, Learning Materials, Activities and Assessments, and Course Policies. These categories offer a comprehensive framework for integrating AI while addressing students’ concerns and enhancing learning experiences.
Course Policies: Establish Clear Guidelines for AI Usage
Reflecting Recommendation 1, developing a clear, transparent policy on AI usage is key. Faculty should articulate when and how students can use AI tools, providing specific examples of ethical use. By defining these expectations early in the course, instructors help students understand the role AI can play in their learning process, promoting academic integrity.
Learner Profiles: Address Emotional and Academic Concerns
In line with Recommendation 2, it is essential to consider students’ diverse reactions to AI—ranging from excitement to anxiety—when designing a course. This is where understanding Learner Profiles becomes critical.
Learning Materials and Activities: Ensure Relevance and Adaptability with AI
Recommendation 3 emphasizes the importance of educating students about generative AI, which can be achieved through thoughtful integration into learning materials, activities, and assessments.
Course Learning Outcomes: Integrate AI with Intentional Learning Design
The integration of generative AI tools into course design necessitates an examination of their impact on student mastery of the Course Learning Outcomes. It is vital to ensure that student use of AI tools supplement and enhance the learning process rather than bypass cognitive engagement.
With these four considerations in mind, we can now introduce a tool to help assess and improve course resilience against generative AI, while providing learners with clear policy decisions and explanations.
Introducing CART: Course AI Resiliency Tracker
In response to the clear need for effective integration of generative AI in educational settings, a new tool has been developed (as part of a wider suite of artificial intelligence tools) to assist faculty in navigating this complex landscape. This tool is designed to support instructors in evaluating how generative AI could respond to their course learning outcomes by highlighting its current capabilities to address and complete these outcomes. It facilitates a detailed understanding of learner profiles to ensure that AI applications are relevant and accessible to all students. Additionally, the tool encourages faculty to reflect on the currency and relevance of their learning materials and to assess how AI might be incorporated into activities and assignments. By examining existing course policies on AI usage and offering actionable steps for course development, this resource aims to demystify generative AI for both educators and students, promoting a thoughtful and strategic approach to its integration or decision to restrict AI.
Getting Started
Upon accessing the landing page, you will be prompted to input your Course ID, after which you may proceed by selecting the “Start” button.
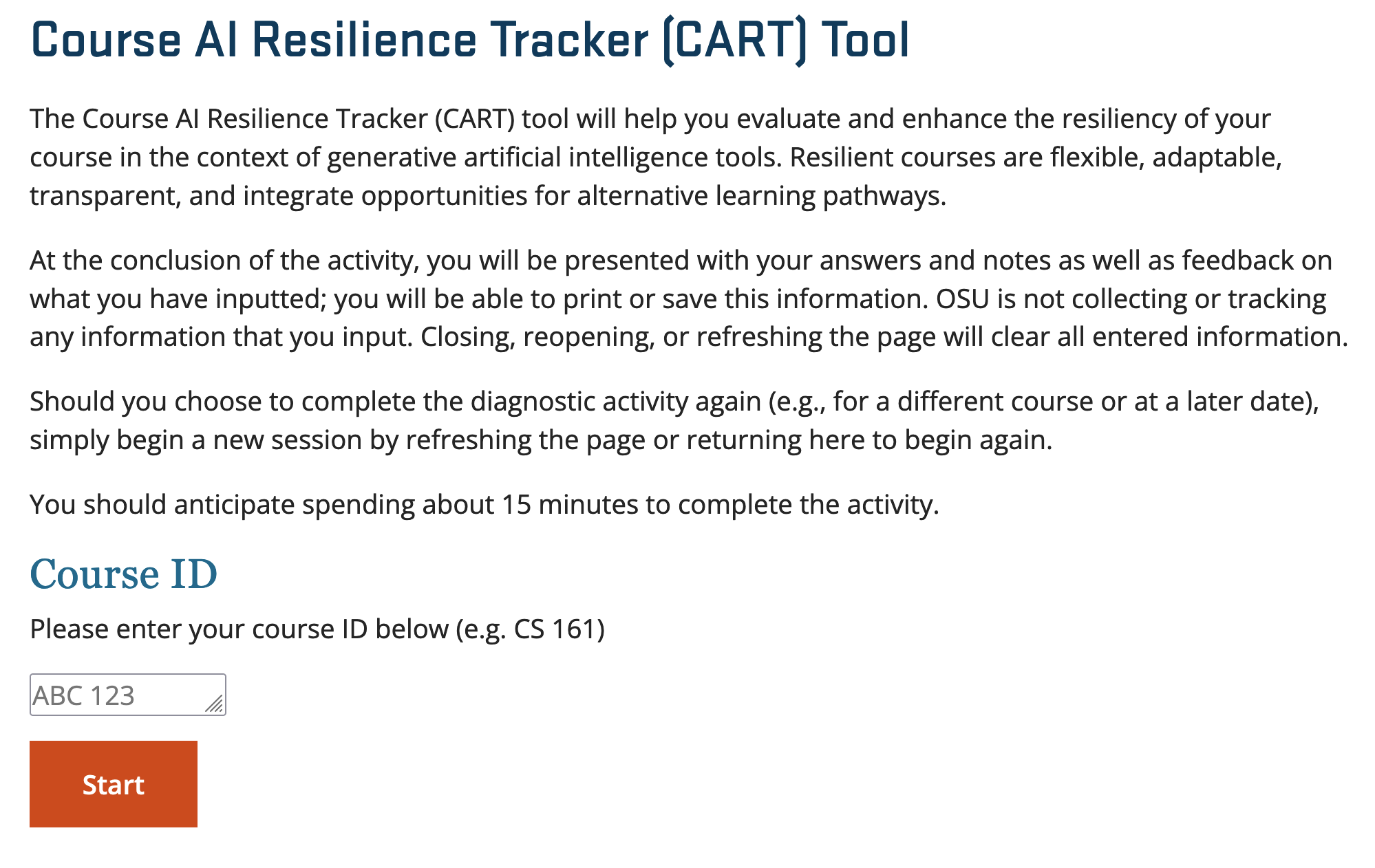
Learning Outcomes
The first step in the tool involves a reflection on your Course Learning Outcomes (CLOs). At this stage, you will have the option to choose from a list of commonly used learning outcome verbs, organized by the general categories of Bloom’s Taxonomy. Note that there is a current selection limit of five CLOs at one time, and faculty with verbs absent from this list are encouraged at this time to select verbs that are most like those in their own CLOs to get feedback that will feel the most transferable.
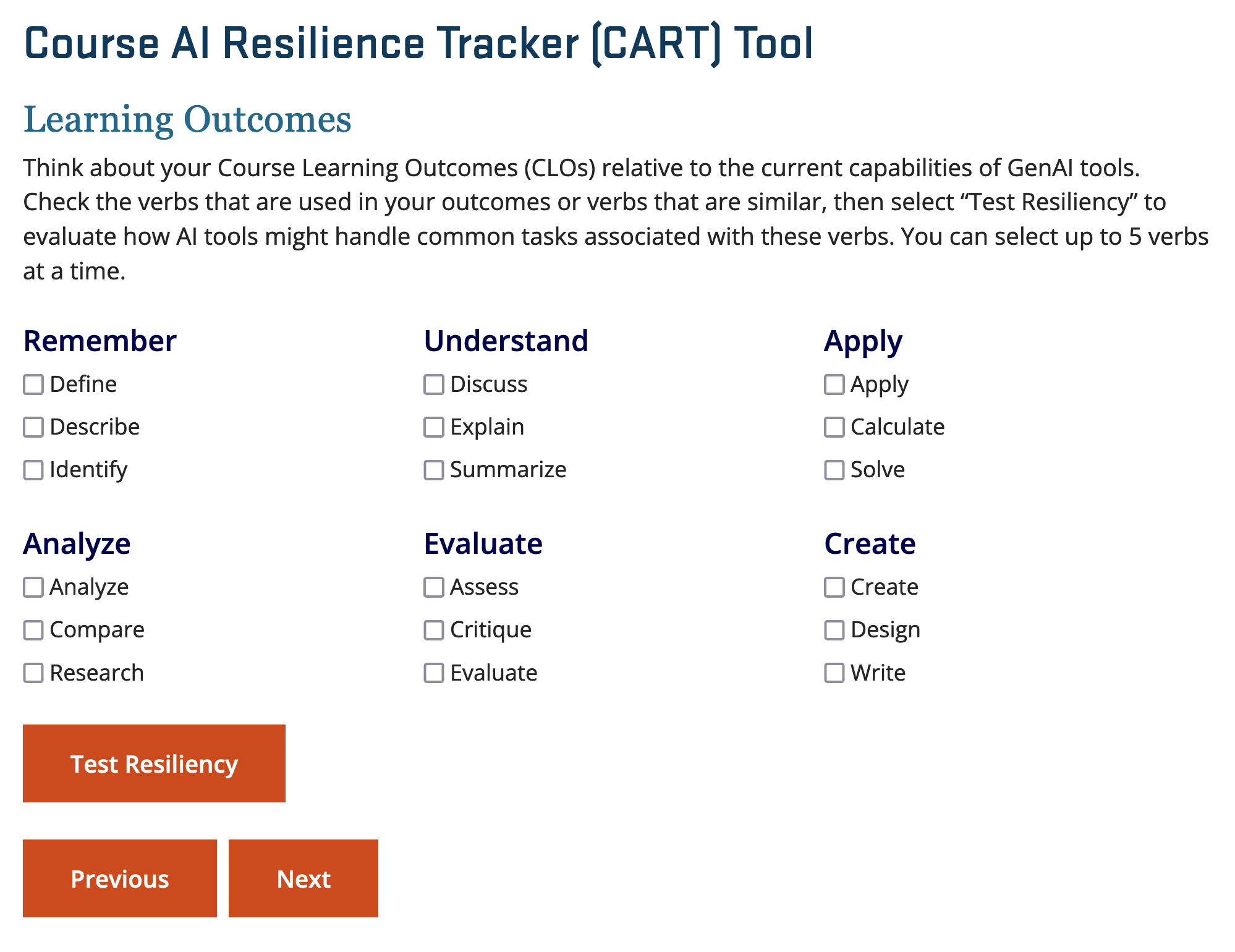
After selecting the appropriate verbs that align with your outcomes, click on the “Test Resiliency” button. This will display feedback on how generative AI may already be able to meet expectations for common tasks associated with those action verbs.
Your Learners
Following the assessment of CLOs, the next step encourages you to consider your learners. In this section, you are invited to input relevant details about your students, including their backgrounds, career aspirations, prior knowledge, or any other contextual information that could inform your generative AI course policies. We are aware that this question might feel challenging, especially for faculty who teach all kinds of learners as part of a general education course. In this case, consider this as a more general introduction to the wide variety of learner profiles that may take the course, and how generative AI may be used from their perspective.
Your responses here, as with all inputs in the tool, will be temporarily stored and displayed on the Summary Page for your future reference.
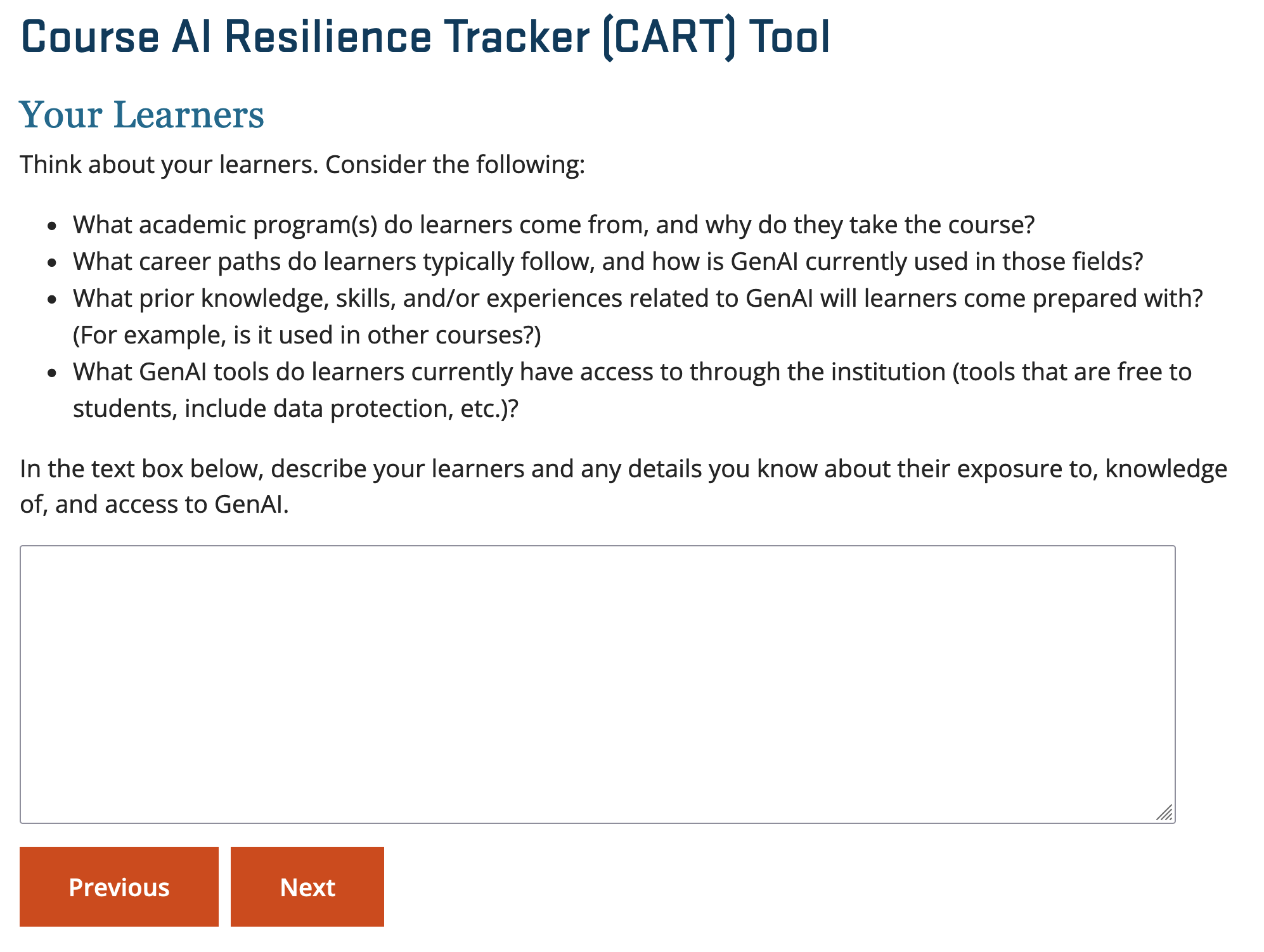
Learning Materials
Next, the tool asks you to evaluate the relevance and adaptability of your learning materials. You may choose from the pre-set options provided, or alternatively, you can select “Other” to add customized choices based on your specific course materials.
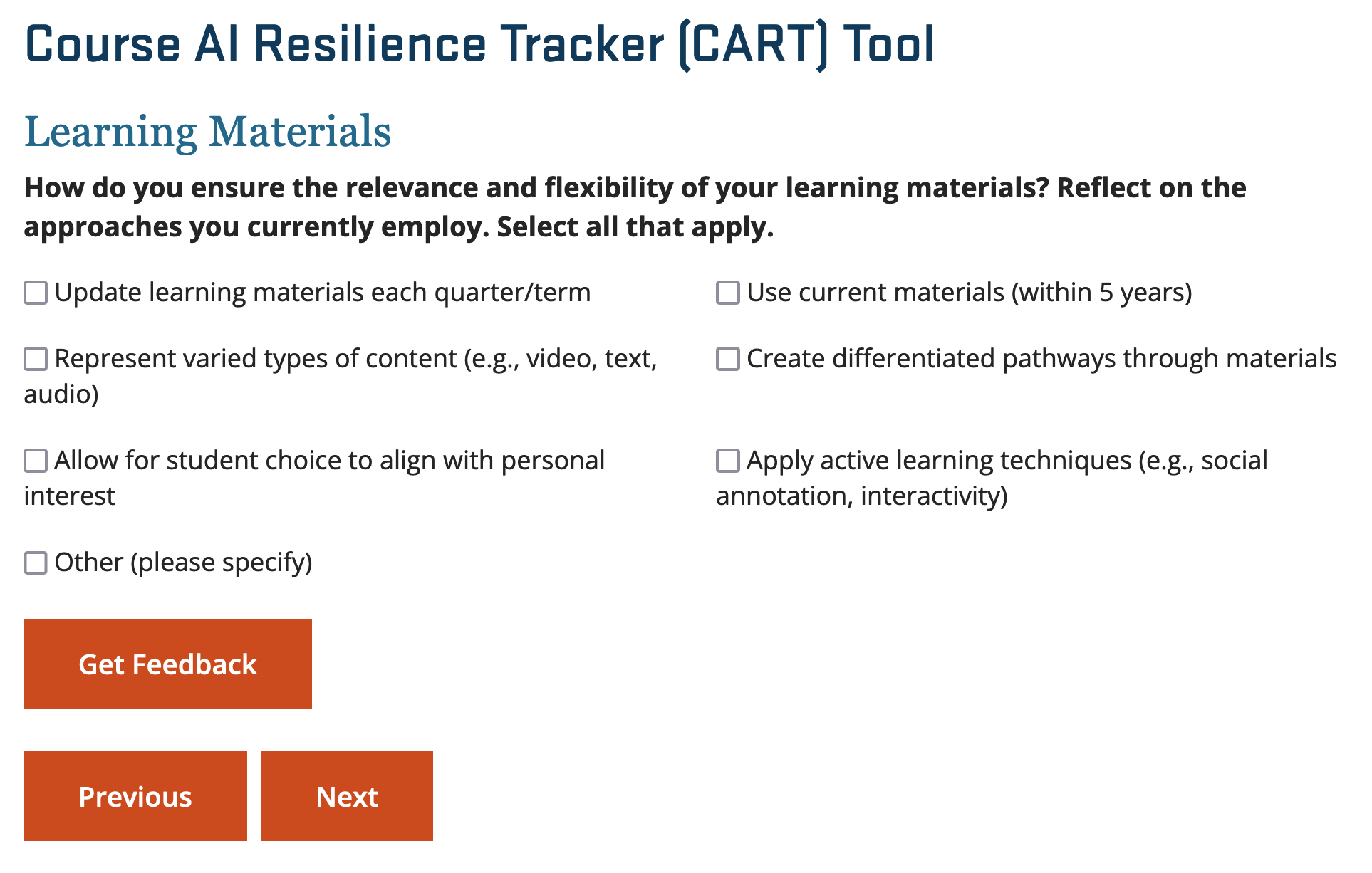
Activities and Assessments
Next, you will be prompted to reflect on your course activities and assessments. This section includes three key questions. Two of the questions are straightforward yes-or-no inquiries, while the third invites you to select one or more methods that you currently employ to promote academic integrity in your assessments. Including this information alongside activities and assessments bolsters understanding for your learners about expected Gen AI usage, why the choice has been made, and enhances academic integrity across the entire course.
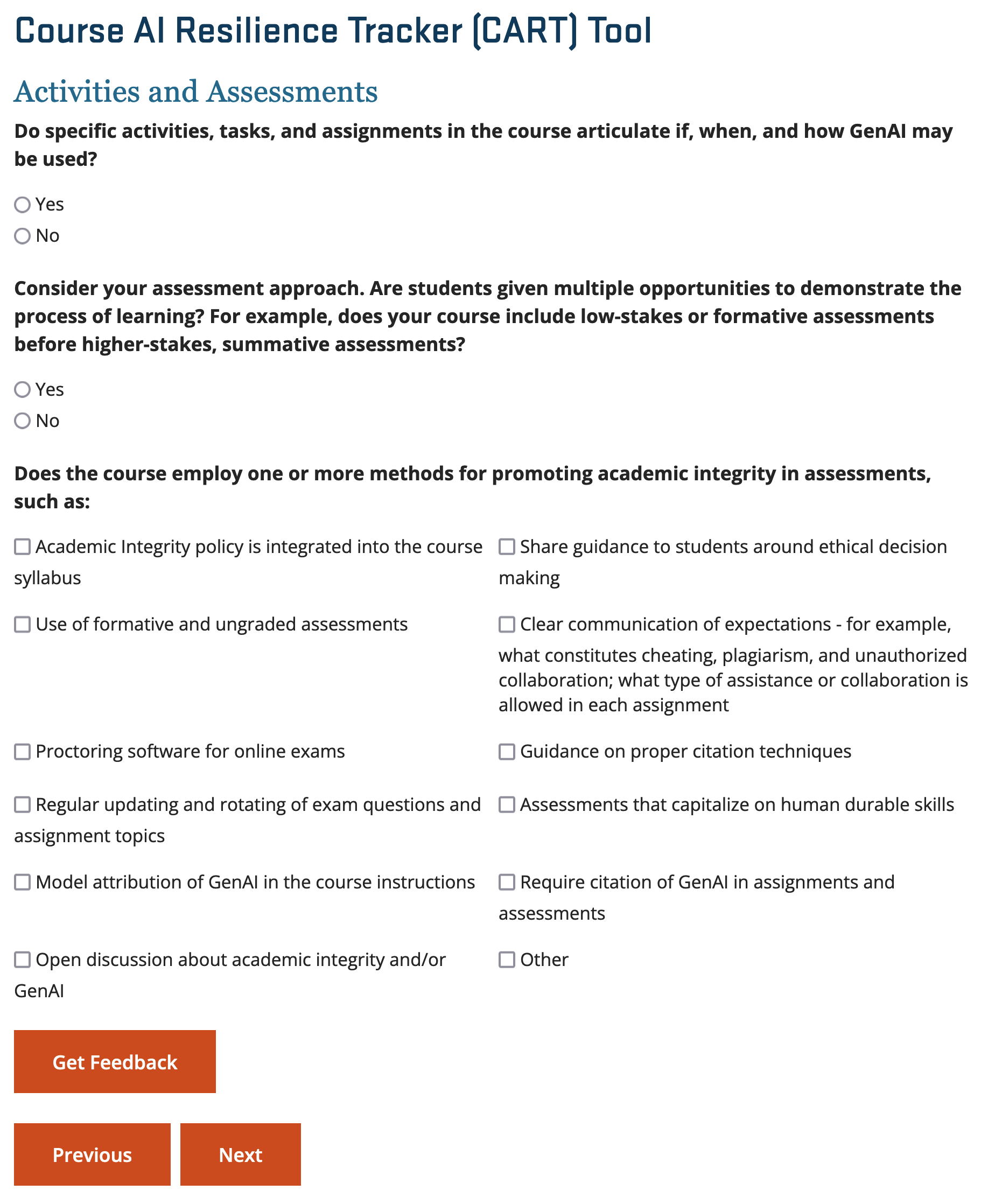
Course Policies
You will then be prompted to consider an important question: does your syllabus currently include a policy on generative AI? This reflection is crucial for ensuring transparency and consistency in how AI is addressed throughout your course design. After choosing one of the answers, you will be able to select from some key elements to include in your AI usage policy.
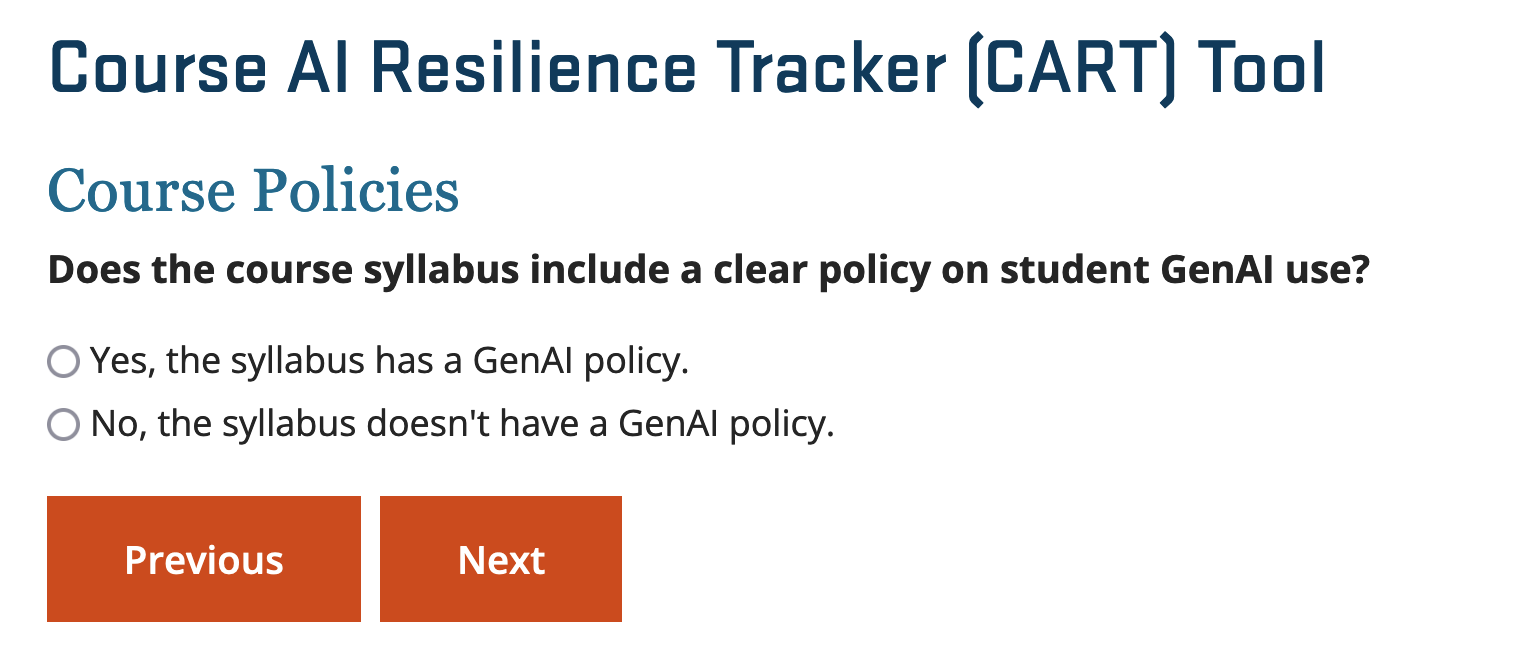
Next Steps
Finally, the tool concludes by prompting you to consider the next steps in your course development, offering guidance on how to proceed with integrating generative AI effectively. Each choice offers different recommendations as automatic feedback, and you are encouraged to read through them all before moving onto the final summary.
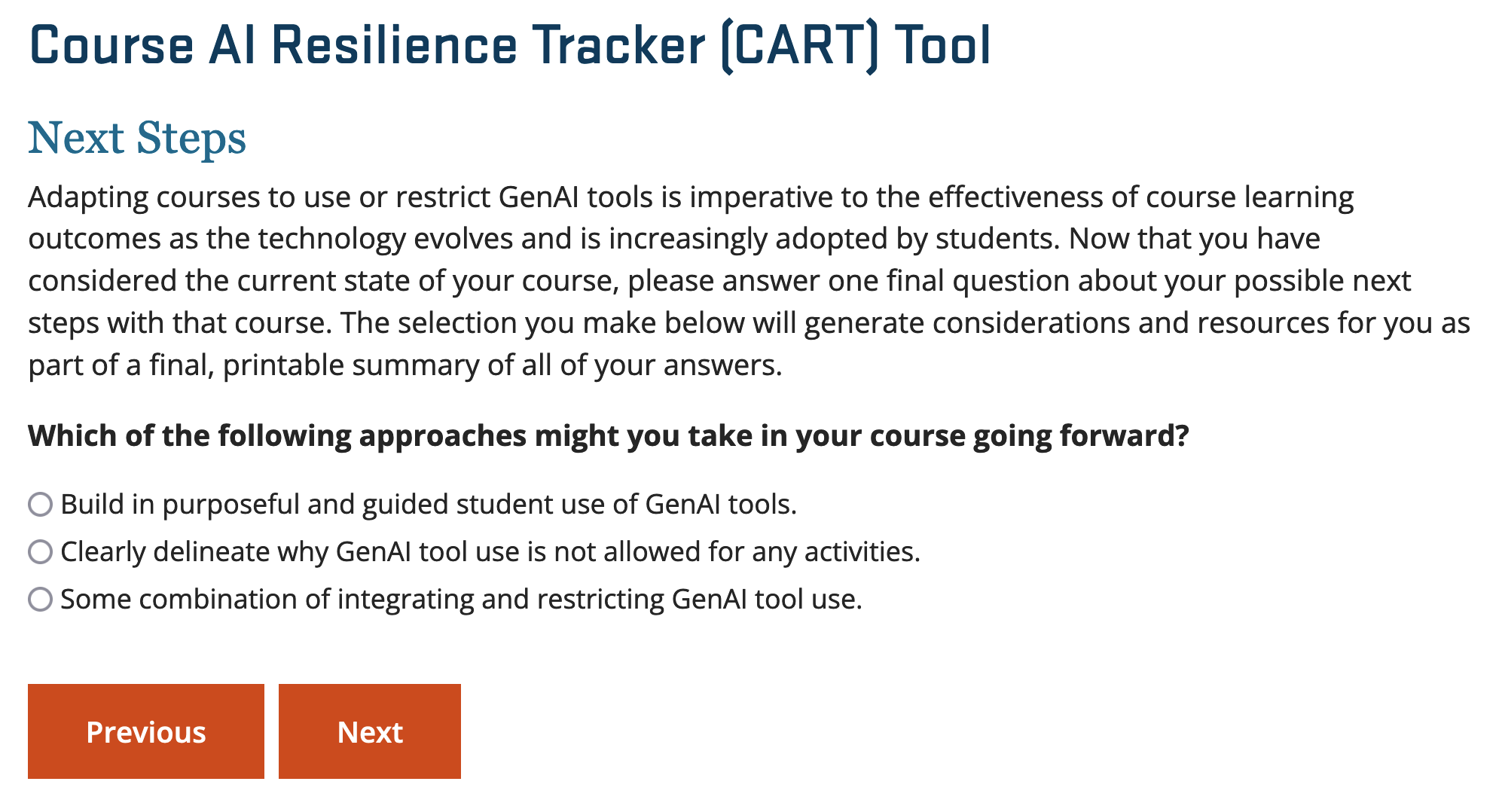
Summary Page
At the conclusion of the tool, you will be directed to a Summary Page that consolidates all your previous inputs, along with the guidance and recommendations provided throughout the process. This comprehensive summary can be printed or saved as a PDF for future reference and review.
The benefits of using the tool
Recommendation 1: A clearly explained course policy
The new tool supports this recommendation by guiding instructors to design course policies that offer clear instructions to learners on what is allowed and disallowed, and most importantly to give rationales behind these policy decisions.
Recommendation 2: Considering learner profiles
The tool helps instructors map these profiles to ensure that generative AI is integrated in ways that are accessible, equitable, and aligned with the emotional and cognitive needs of different students. By anticipating student concerns, instructors can provide thoughtful guidance on how AI will or will not be used in various course activities and assessments.
Recommendation 3: Ensure Relevance and Adaptability with AI
The tool helps instructors evaluate the relevance and adaptability of their current materials by offering pre-set options or the ability to add customized choices. This process ensures that course content remains up-to-date and flexible enough to incorporate generative AI effectively or alternatively, provides avenues to secure assessments against AI generated content.
Course Learning Outcomes: Integrate AI with Intentional Learning Design
The tool supports this by guiding instructors through a reflection on their CLOs, offering a selection of commonly used learning outcome verbs categorized by Bloom’s Taxonomy. It also helps educators recognize the extent to which generative AI can currently accomplish many of these learning outcomes, providing valuable insights into the specific areas where AI might enhance or support course goals. the purpose of this is to ensure that AI integration choices are not just incidental, but strategically aligned with fostering critical thinking, creativity, and problem-solving skills within the broader context of your course objectives.
Conclusion
In response to the growing need for effective AI integration, this new tool helps faculty navigate the complexities of incorporating generative AI into course design. By addressing Course Learning Outcomes, Learner Profiles, Learning Materials, Activities and Assessments, and Course Policies, the tool promotes a strategic approach that aims to demystify AI for both educators and students. With thoughtful integration, well-designed generative AI policies can enhance learning experiences, help prepare students for future, teach learners to avoid potential pitfalls, and maintain the academic integrity of online courses.
License and Attribution
License
Course AI Resilience Tracker Tool, created by Oregon State University Ecampus, is licensed under Creative Commons Attribution-NonCommercial 4.0 International
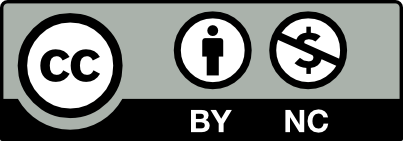
Text Content and Guidance
Ashlee Foster, Dana Simionescu, Philip Chambers, Katherine McAlvage, and Cub Kahn
HTML/JavaScript Development
Philip Chambers
References
Dello Stritto, M. E., Underhill G. R., & Aguiar, N. R. (2024). Online Students’ Perceptions of Generative AI. Oregon State University Ecampus Research Unit. https://ecampus.oregonstate.edu/research/publications/