Introduction
We’ve all heard by now of ChatGPT, the large language model-based chat bot that can seemingly answer most any question you present it. What if there were a way to provide this functionality to students on their learning management system, and it could answer questions they had about course content? Sure, this would not completely replace the instructor, nor would it be intended to. Instead, for quick course content questions, a chatbot with access to all course materials could provide students with speedy feedback and clarifications in far less time than the standard turnaround required through the usual channels. Of course, more involved questions about assignments and course content questions outside of the scope of course materials would be more suited to the instructor, and the exact usage of a tool like this would need to be explained, as with anything.
Such a tool could be a useful addition to an online course because not only could it potentially save a lot of time, but it could also keep students on the learning platform instead of using a 3rd-party solution to answer questions as is the suspected case right now with currently available chatbots.
To find out what this would look like, I researched a bit on potential LLM chatbot candidates, and came up with a plan to integrate one into a Canvas page.
Disclaimer!
This is simply a proof of concept, and is not in production due to certain unknowns such as origin of the initial training data, CPU-bound performance, and pedagogical implications. See the Limitations and Considerations section for more details.
How it works
The main powerhouse behind this is an open source, Large Language Model (LLM) called privateGPT. privateGPT is designed to let you “ask questions to your documents” offline, with privacy as the goal. It therefore seemed like the best way to test this concept out. The owner of the privateGPT repository, Iván Martínez, notes that privacy is prioritized over accuracy. To quote the ReadMe file from GitHub:
100% private, no data leaves your execution environment at any point. You can ingest documents and ask questions without an internet connection!
privateGPT, GitHub Site
privateGPT, at the time of writing, was licensed under the Apache-2.0 license, but during this test, no modifications were made to the privateGPT code. Initially, when you run privateGPT, train it on your documents, and ask it questions, you are doing all of this locally through a command line interface in a terminal window. This obviously will not do if we want to integrate it into something like Canvas, so additional tools needed to be built to bridge the gap.
I therefore set about making two additional pieces of software: a web-interface chat box that would later be embedded into a Canvas page, and a small application to connect what the student would type in the chat box to privateGPT, then strip irrelevant data from its response (such as redundant words like “answer” or listing the source documents for the answer) and push that back to the chat box.

Once created, the web interface portion, running locally, allows us to plug it into a Canvas page, like so:
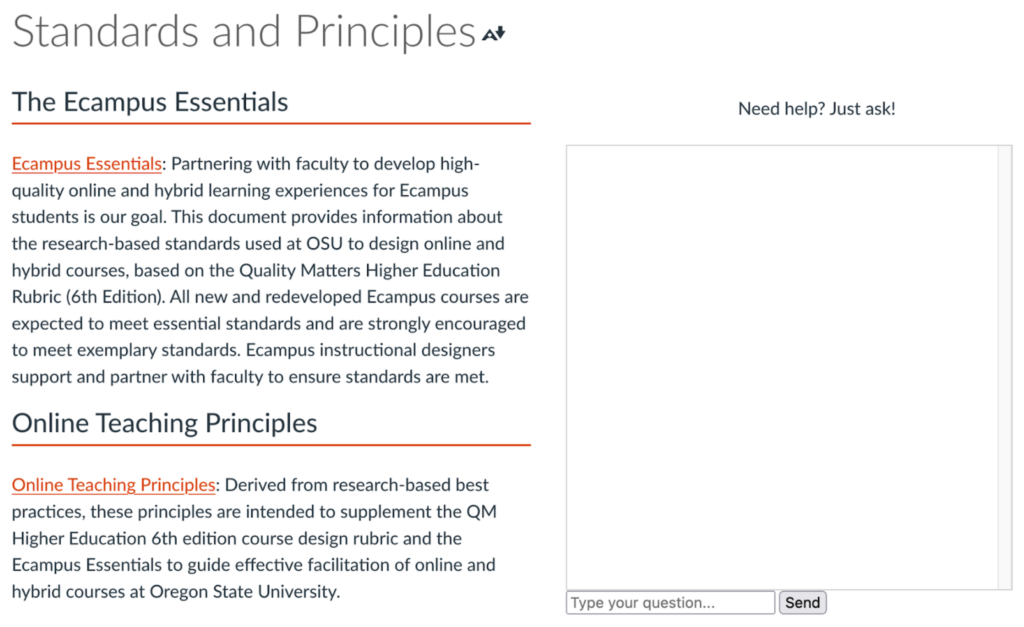
Testing how it works
To begin, I let the LLM ‘ingest’ the Ecampus Essentials document provided to course developers on the Ecampus website. Then I asked some questions to test it out, one of which was: “What are the Ecampus Essentials?”
I am not sure what I expected here, as it is quite an open ended question, only that it would scan its trained model data and the ingested files looking for an answer. After a while (edited for time) the bot responded:
A successful result! It has indeed pulled text from the Ecampus Essentials document, but interestingly has also paraphrased certain parts of it as well. Perhaps this is down to the amount of text it is capable of generating, along with the model that was initially selected.
A longer text example
So what happens if you give it a longer text, such as an OpenStax textbook? Would it be able to answer questions students might have about course content inside the book?
To find out, I gave the chatbot the OpenStax textbook Calculus 1, which you can download for free at the OpenStax website. No modifications were made to this text.
Then I asked the chatbot some calculus questions to see what it came up with:
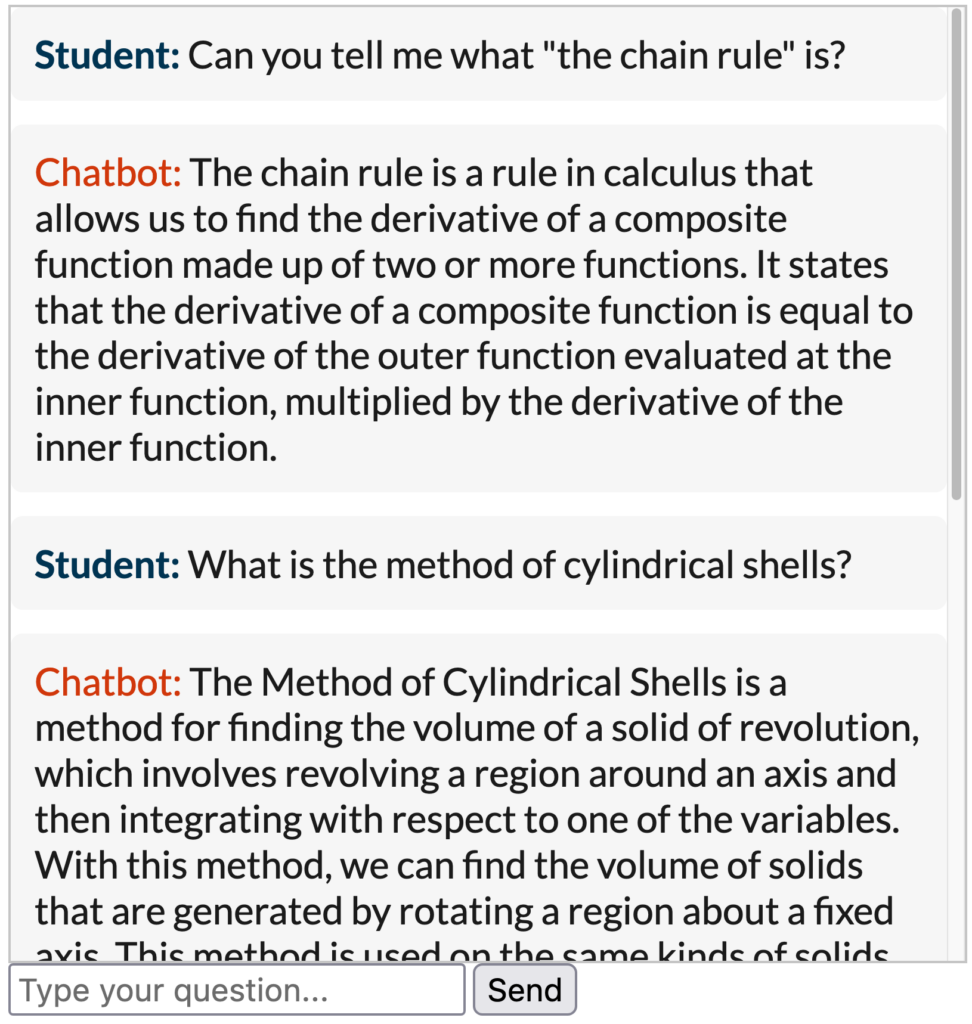
It would appear that if students had any questions about mathematical theory, they could get a nice (and potentially accurate) summary from a chatbot such as this. Though this brings up some pedagogical considerations such as: would this make students less likely to read textbooks? Would this be able to search for answers to quiz questions and/or assignment problems? It is already common to ask ChatGPT to provide summaries and discussion board replies, would this bot function in much the same way?
Asking the chatbot to calculate things, however, is where one would run into the current limitations of the program, as it is not designed for that. Simple sums such as “1 + 1” return the correct answer, as this is part of the training data or otherwise common knowledge. Asking it to do something like calculate the hypotenuse of a triangle using Pythagorus’ theorem will not be successful (even using a textbook example of 32 + 42 = c2). The bot will attempt to give an answer, but its accuracy will vary wildly based on the data given to it. I could not get it to give me the correct response, but that was expected as this was not in the ingested documentation.
Limitations and Considerations
OK, so it’s not all perfect – far from it, in fact! The version of privateGPT I was using, while impressive, had some interesting quirks in certain responses. Responses were never identical either, but perhaps that is to be expected from a generative LLM. Still, this would require further investigation and testing in a production-ready model.
How regular and substantive interaction (RSI) might be affected is an important point to consider, as a more capable chatbot could impact the student-instructor Q&A discussion board side of things without prior planning on intended usage.
A major technical issue was that I was limited to using the central processing unit (CPU) instead of the much faster graphics processing unit (GPU) used in other LLMs and generative AI tools. This meant that the time between the question being sent and the answer being generated was far higher than desired. As of writing, there appears to be a way to switch privateGPT to GPU instead, which would greatly increase performance on systems with a modern GPU. The processing power required for a chatbot that more than one user would interact with simultaneously would be substantial.
Additionally, the incorporation of a chatbot like this has some other pedagogical implications, such as how the program would respond to questions related to assignment answers, which would need to be researched.
We also need to consider the technical skill required to create and upkeep a chatbot. Despite going through all of this, I am no Artificial Intelligence or Machine Learning expert; a dedicated team would be required to maintain the chatbot’s functionality to a high-enough standard.
Conclusion
In the end, the purpose of this little project was to test if this could be a tool students might find useful and could help them with content questions faster than contacting the instructor. From the small number of tests I conducted, it is very promising, and perhaps a properly built version could be used as a private alternative to ChatGPT, which is already being used by students for this very purpose. A major limitation was running the program from a single computer with consumer components made 3 years ago. With modern hardware and software – perhaps a first-party integrated version built directly into a learning management system like Canvas – students could be provided with their own course- or platform-specific chatbot for course documents and texts.
If you can see any additional uses, or potential benefits or downsides to something like this, leave a comment!
Notes
- Martínez Toro, I., Gallego Vico, D., & Orgaz, P. (2023). PrivateGPT [Computer software]. https://github.com/imartinez/privateGPT.
- “Calculus 1” is copyrighted by Rice University and licensed under an Attribution-NonCommercial-Sharealike 4.0 International License (CC BY-NC-SA).