A major part of research in our group is developing methods to predict the complex nearshore ocean, often by combining observational data with models. Data assimilation is one way to optimally combine observations with a model prediction to produce an overall better estimate of the true ocean state.
Model-Data Assimilation With Tagged Coastal Seabirds: One of our ongoing data assimilation projects is the Cormorant Oceanography Project, funded by the Office of Naval Research as the DASHCAMS directed research initiative. Working with seabird ecologists, the project is using small sensors attached to diving seabirds (cormorants) to collect oceanographic data. Our group is using data assimilation to translate the broadly-distributed tag data into oceanographic predictions of bathymetry and nearshore currents. You can learn more about the overall project from this article in EOS Magazine.
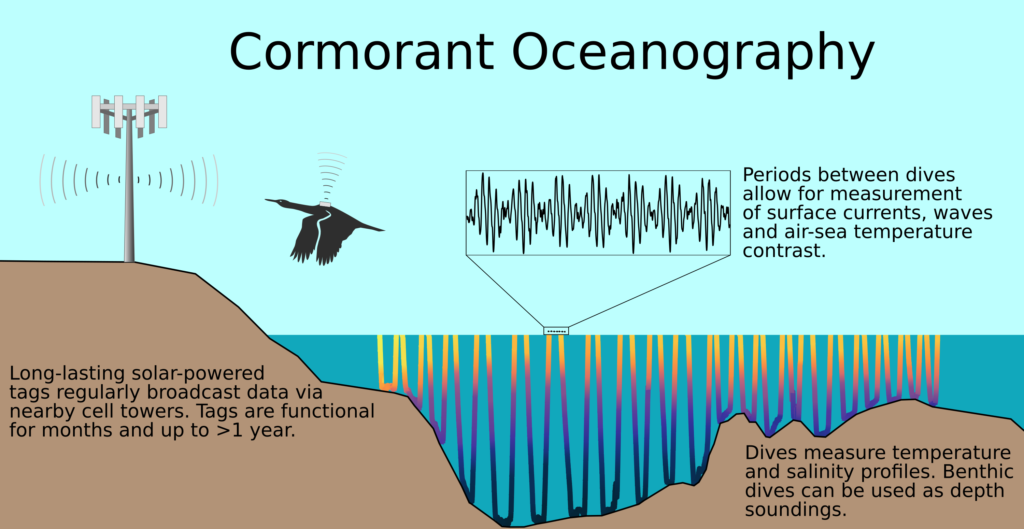
Surfzone Model Uncertainty Quantification: The US Army Corps of Engineers has developed an exciting Coastal Model Test Bed (CMTB), which aims to make model validation and inter-comparisons more widespread in the nearshore community by leveraging multiple codes and data sources. In collaboration with USACE scientists and with funding from the US Coastal Research Program, we are transitioning our research codes for data assiimlation and uncertainty quantification into the CMTB. A major part of this effort is to better represent model uncertainty, especially that of bathymetry, in nearshore forecasts.
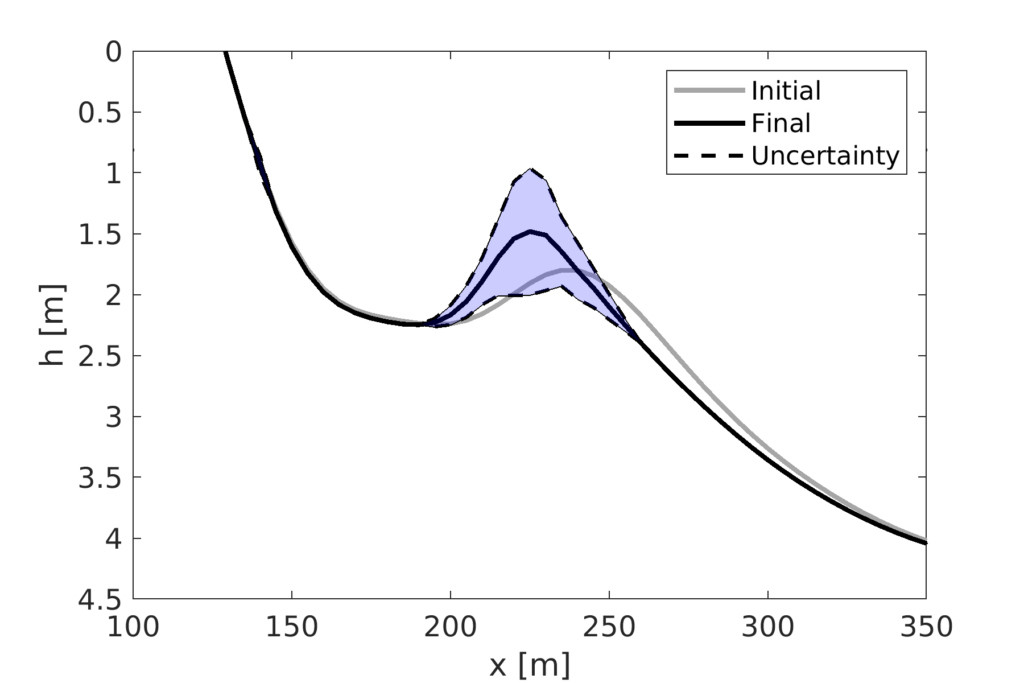
Coastal Image Analysis: We are also exploring new methods to analyze coastal imagery for long-term morphology. Former PhD student Ashley Ellenson developed a new application of deep-learning to detect typical beach “states” important to nearshore morphodynamics, based on 30 years of Argus beach camera images from Duck NC and Narrabeen Australia. The results of Ashley’s work can be seen in this paper and github repo.
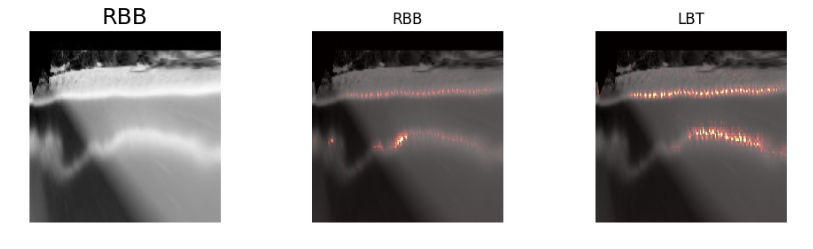