Caitlin Barbour, GEOG 560 Oregon State University, Fall 2020
In recent years wildfires have continued to grow in size and frequency as the effects of fire suppression practices have accumulated. To better view the affects of wildfires on the landscape and how they impact nearby communities, it is important to be able to assess potential risks regarding fuels, topography, weather patterns, and public safety. In this literature review, the techniques used to identify and classify variables and how they are analyzed to map risks are addressed.
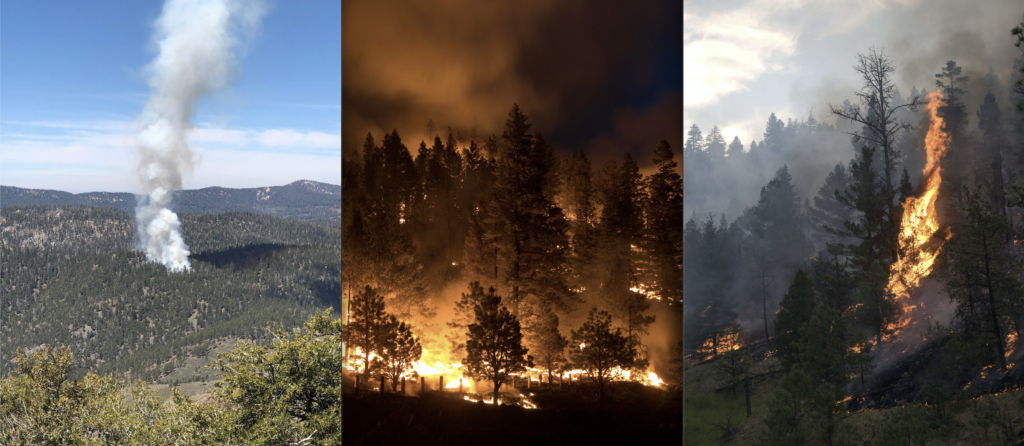
Annotated Bibliography
Craig Delong, S., and David Tanner. 1996. “Managing the Pattern of Forest Harvest: Lessons from Wildfire.” Biodiversity and Conservation 5 (10): 1191–1205. doi:10.1007/BF00051571.
Current forest management practices entail that harvesting mimics natural disturbance events, however this pattern does not match with the historic patterns created by wildfire when spatial characteristics are compared. These patterns are comprised of individual wildfires, habitat types, and the landscape as a whole. This article worked on the hypotheses that wildfires were becoming rarer and smaller with clearcutting being the dominant disturbance event. With these hypotheses and the differences in spatial patterns it was found that the age of the forest harvested is more selective (usually older) than the age of the forest disturbed by wildfire. It is proposed that harvesting should be allowed beyond the 80ha limit to lower the area disturbed by wildfire.
Hysa, Artan, and Fatma Ayçim Türer Başkaya. 2019. “A GIS Based Method for Indexing the Broad-Leaved Forest Surfaces by Their Wildfire Ignition Probability and Wildfire Spreading Capacity.” Modeling Earth Systems and Environment 5 (1): 71–84. doi:10.1007/s40808-018-0519-9.
Proximity to areas of human urbanization has been shown to increase the potential for wildfire ignition. However, most ignition points occur where residency is less dense and vegetation is more dense. Through inventory of forest cover, analysis of that inventory, and mapping the WIPI and WSCI indexes a model can be generated to identify ignition probably and impact capacity. Hysa et al approaches this issue by differentiating ignition and capacity of a wildfire as it is most effective when temporal data for a region is limited. This differentiating, via analytical weighting, provides an insight to how forest surfaces are spatially distributed based on various criteria such as roads, water resources, urbanization, and agriculture. According to these various classifications, the distances between the ignition points and the criteria classifications are consistent and match with prior burn locations.
Kalabokidis, Kostas, Alan Ager, Mark Finney, Nikos Athanasis, Palaiologos Palaiologou, and Christos Vasilakos. 2016. “AEGIS: A Wildfire Prevention and Management Information System.” Natural Hazards and Earth System Sciences 16 (3): 643–661. doi:10.5194/nhess-16-643-2016.
Coordination, control, planning, and getting early warnings of wildfires is essential to wildfire management and Greece has been assisted with this through the AEGIS application. AEGIS allows for users to provide spatial and non-spatial data as inputs for modeling of wildfire behavior. Such data are considered to be field inventories and includes fire duration, ignition points, and current weather reports. AEGIS also collects data from other sources detailing infrastructure, roads, topography and more. All this combined allows for the program to model fire behavior and associated hazards through the MTT algorithm in conjunction with FlamMap5. Once the model has been built, AEGIS allows access of the layers to other users to create their own simulations. This way of providing simulation modeling to all users allows for the general shape and direction of wildfire to be anticipated and allows for additional what-if type questions to be tested.
Kalabokidis, Kostas D, Pavlos Konstantinidis, and Christos Vasilakos. 2002. “GIS Analysis of Physical and Human Impact on Wildfire Patterns,” 13.
Human activity is an important factor to account for when analyzing wildfire patters, along with the regular factors of vegetation, soil, and weather distribution and patterns. The human element provides a look at how the human population interacts with the landscape from grazing practices to other modifications including roads and facilities. Through the use of GIS, a thematic map can be produced that isolates the various elements of human activity on the landscape to calculate correlations between their spatial distributions. By calculating these correlations, the authors were able to create thematic maps that utilized 1and 0 values for burned and unburned units and the same values for units with vegetation and without vegetation. Additional model analysis shows that human activity is significant in areas where vegetation has been cultivated over a long period of time and these areas also show degradation to the non-cultivated vegetation. This activity affects wildfire patterns by influencing wildfire direction and intensity due to the presence of artificial modifications and non-fire adapted vegetation being cultivated.
Keane, Robert E., Robert Burgan, and Jan van Wagtendonk. 2001. “Mapping Wildland Fuels for Fire Management across Multiple Scales: Integrating Remote Sensing, GIS, and Biophysical Modeling.” International Journal of Wildland Fire 10 (4): 301. doi:10.1071/WF01028.
Knowing how fires will act and spread based on the fuel load and distribution on the landscape is important to assessing risk especially as suppression has been prominent for many decades. There are different fuel classifications (loading, size, and density) and the locations of these fuels (ground or crown) that affect how severe and intense the wildfires will be. With fire suppression fuel loads have increased and are predominant among tree crowns. To more accurately describe these fuel types, a fuel model is used that helps to predict wildfire behavior and from which fuels can be mapped. This mapping can occur through four different methods which are field reconnaissance, direct and indirect mapping through remote sensing and combining environmental gradients and biophysical modeling. Each of these methods has their strengths, from mapping actual ground conditions to streamlining the process, to frequency of use allowing for ease of updating, and to applying ecological perspectives to the fuel loads. The most beneficial method is the field sampling as it provides on-site current data, however it can be buffered by being blended with the other methods. This allows for the development of a comprehensive fuel model that allows or imagery to supplement the field data and create simulations for management to develop strategies.
Li, Dapeng, Thomas J. Cova, and Philip E. Dennison. 2019. “Setting Wildfire Evacuation Triggers by Coupling Fire and Traffic Simulation Models: A Spatiotemporal GIS Approach.” Fire Technology 55 (2): 617–642. doi:10.1007/s10694-018-0771-6.
With WUIs prominence in the Wester US public safety is paramount and evacuations are necessary to ensure the safety of the public and efficiency of wildfire management practices. Traffic simulations and using topography to identify safety points in relation to predicted fire behavior allows for evacuation plans to be planned and modified as fires occur. By using spatiotemporal GIS, the time it takes for wildfire spread to occur can also be factored into evacuation plans. Simulation models that analyze the relationship between the ignition point, community location, the buffer zone, and weather patterns provide timing estimates for evacuation and ways of providing warnings based on risk assessment. Such scenarios are important for high-fire risk and low-egress communities as many are far from emergency services and methods of entry are few or slow.
Mahdavi, A, S R Fallah Shamsi, and R Nazari. 2011. “Forests and Rangelands’ Wildfire Risk Zoning Using GIS and AHP Techniques.” Caspian Journal of Environmental Sciences 10 (1).
Wildfires are considered ecological disasters and risk zoning provides a way for more effective conservation efforts. Mapping these risk zones is done by utilizing DEM, population, temperature and precipitation in ArcGIS. Landcover was taken from imagery that was derived from both digital classification and visual interpretation. The AHP procedure was used to weight these factors against each other. These variables, once entered into ArcGIS for analysis, showed that the higher risk zones matched with actual fires that had occurred and was an important correlation to occur in this testing of risk zoning.
Oliveira, Sandra, Francisco Moreira, Roberto Boca, Jesús San-Miguel-Ayanz, and José M. C. Pereira. 2014. “Assessment of Fire Selectivity in Relation to Land Cover and Topography: A Comparison between Southern European Countries.” International Journal of Wildland Fire 23 (5): 620. doi:10.1071/WF12053.
Wildfire behavior is influenced by topography, vegetation, weather variables, fuel loads and types, as well as land utilization. Oliveira et al. looks into how different landscapes affect wildfire frequency by assessing the type of landcover according to the CLC database and burnt areas greater than 40 ha. The relationship between the two is then assessed in relation to topography through either topographic category, land cover type against topography, or topography against land cover type. The results show that the selectivity of wildfires is more prone in areas that are more sparsely vegetative, grassland, and shrubland while agriculture zones are less preferred. The effects of topography on this selection are based on ease of fire being able to climb the slopes due to accessibility of fuel material.
Paveglio, Travis B., Catrin M. Edgeley, and Amanda M. Stasiewicz. 2018. “Assessing Influences on Social Vulnerability to Wildfire Using Surveys, Spatial Data and Wildfire Simulations.” Journal of Environmental Management 213 (May): 425–439. doi:10.1016/j.jenvman.2018.02.068.
Loss of economy, injury, destruction of property are among the many concerns of wildfire impacts to residential areas. Pavelgio et al. approaches this issue by looking at how population vulnerability changes based on sociodemographic characteristics, support of local wildfire management and mitigation practices, how residents perceive their risk level, and the relationship to parcel-specific properties. Four models were run with in relation to wildfire exposure risk, wildfire sensitivity, fuel levels in the Home Ignition Zone, and property values. It was found that residents who selected their property based on wildfire risk was the most consistent variable where the inconsistent variables included property age and development. Wildfire risk is higher in developments recently built in proximity to wildland vegetation while residences of higher-value are at higher risk for exposure and sensistivity. Through these model simulations Pavelgio et al determined that wildfire risk is lower for those closer to city centers and that fine-scale measures would be needed for specific local results.
Polychronaki, A., I. Z. Gitas, and A. Minchella. 2014. “Monitoring Post-Fire Vegetation Recovery in the Mediterranean Using SPOT and ERS Imagery.” International Journal of Wildland Fire 23 (5): 631. doi:10.1071/WF12058.r 14
Monitoring of post-fire vegetation recovery is frequently utilized through the traditional methods of vegetation indices and imagery. Using SPOT and ERS imagery, the images were orthorectified before being correction through the COST method. After being processed the imagery is matched to land-cover types, of which six were created based on imagery from 2007 and 2008. Once land-cover type and scale are determined, the images are analyzed for statistics and multi-temporal backscattering in post-fire areas. Through this process it was found that in order to differentiate between forest and dense shrub vegetation, a matrix was run that did not include SAR from the ERS images, allowing shadowed areas to be located. Additionally, it was revealed that while little of the forests had regenerated 20 years post-burn and those that did were the north and north-west-facing areas and many of the low vegetation areas had been taken over by shrub.
Pultar, Edward, Martin Raubal, Thomas J Cova, and Michael F Goodchild. 2009. “Dynamic GIS Case Studies: Wildfire Evacuation and Volunteered Geographic Information.” Transactions in GIS 13 (June): 85–104. doi:10.1111/j.1467-9671.2009.01157.x.
Not only is identifying and managing a wildfire important, but being able to assess public safety and incorporate crowdsourced data can lead to more effective decisions. Through the use of EDGIS, the authors investigate the wildfire management methods that involve evacuations, remote sensing, crowdsourced data, and distance to safety zones. The process for this analysis involves mapping the STPs that make up the foundation of the wildfire area and other influencing factors. Once the STP structure has been created, a dynamic GIS helps to visualize these points in relation to evacuation polygons in relation to existing data. In addition to the dynamic GIS, crowdsourced data provides another insight to wildfire activity. Crowdsourcing provides a way for travelers and locals to connect and share networks, as shown through the CouchSurfer database. Specifically, this function allows for individuals to react in real time to wildfire activity and highlights a need for wildfire evacuation research.
Romero-Calcerrada, Raul, C. J. Novillo, J. D. A. Millington, and I. Gomez-Jimenez. 2008. “GIS Analysis of Spatial Patterns of Human-Caused Wildfire Ignition Risk in the SW of Madrid (Central Spain).” Landscape Ecology 23 (3): 341–354. doi:10.1007/s10980-008-9190-2.
Human activity plays an important role in wildfire as humans contribute much to the ignition and behavior of the wildfires. The human influence on these risks is most likely driven by urban growth into WUI zones and recreational activities in forested areas. The authors of this article look into the relationship of these factors with wildfire risk by utilizing ignition reports and weighting socio-economic data. There were then overlapped to generalize the evidence of human influence before looking at the evidences’ conditional independence and combining them. This generates a probabilities map that is validated through known, and observed, ignition points. By going through this process, the models show that wildfire presence is affected by season duration, grazing types, and accessibility. Future risk assessments need to include human activity variables for more effective wildfire management practices.
Scott, Joe H., Matthew P. Thompson, and David E. Calkin. 2013. “A Wildfire Risk Assessment Framework for Land and Resource Management.” RMRS-GTR-315. Ft. Collins, CO: U.S.
Due to the long-term lasting effects of wildfires the ability to assess risks, such as size, location, and severity, is important to making decisions about responding, assessing and monitoring the fire. The risk of wildfire is based on the likelihood, intensity, and susceptibility to HVRAs. The questions that managers need to address are about mitigation approaches and the effects and they can do this by taking an analytical approach. This approach to risk assessment includes mapping the relationship between wildfire exposure, effects, HVRA characterization, and a simulation of wildfire activity. These relationships are then used to model risk based on fuel data, historical fire and weather patterns, and the likelihood and intensity of fire before assessing how the outputs effect individual HVRAs according to input from resource specialists. Finally, the individual HVRA risk analysis is used to weigh the risk across all HVRAs in the landscape. This assessment allows for a flexible way to make judgements based on current landscape conditions, identify areas of uncertainty, and more easily update based on changes to the landscape.
Sirca, Costantino, Franco Casula, Christophe Bouillon, Belén Fierro García, Monica Maria Fernández Ramiro, Beatriz Vila Molina, and Donatella Spano. 2017. “A Wildfire Risk Oriented GIS Tool for Mapping Rural-Urban Interfaces.” Environmental Modelling & Software 94 (August): 36–47. doi:10.1016/j.envsoft.2017.03.024.
The Wildland-Urban Interface or Rural-Urban Interface, where individuals reside in contact to vegetation, is being re-approached to bring awareness to the risks of this dynamic through analysis in the software RUImap. This software operates with adjustable parameters, such as vegetation, housing, and buffer distances, by producing analysis on burned areas and ignition points through three methodologies at the regional or local scales. The first RUImap methodology works on the regional-scale with landcover and soil sealing as the input data. This method produces coarse statistical data that recognizes housing density and soil cover typology. The following two RUImap methodologies work on the local-scale with the first focusing on burnable areas based on spatial fuel continuity and the second focusing on housing density and distribution as an indicator of population presence. Through this software, it has been indicted that when housing density decreases efforts to manage the surrounding areas for wildfire risk increases and the highest ignition density was found in the intermediate housing density.
Zhang, Fuquan, Pengcheng Zhao, Shuwen Xu, Yin Wu, Xubing Yang, and Yan Zhang. 2020. “Integrating Multiple Factors to Optimize Watchtower Deployment for Wildfire Detection.” Science of The Total Environment 737 (October): 139561. doi:10.1016/j.scitotenv.2020.139561.
Watchtower systems provide early fire detection through the use of HD video recorders and wireless networks. To optimize this system, the authors suggest exploiting resource redundancies and increase the systems stability to make it more easily adaptable elsewhere. To test the efficiency of this fire risk variables, ignition point and spreading, are identified and analyzed for watchtower visibility. Once visibility has been established, the consistency of detection is analyzed to ensure efficiency and that any redundancies in visibility between watchtower locations stable. The optimal approach for early fire detection is monitor the watchtower network as a whole, rather than each watchtower station on its own. Additionally, the more stations that can be implemented the better the coverage would be in high-risk areas as more than one watchtower would have visibility to the same areas.
Additional Literature
Ambrosia, Vincent G., Sally W. Buechel, James A. Brass, James R. Peterson, Richard H. Davies, Ronald J. Kane, and Steve Spain. 1998. “An Integration of Remote Sensing, GIS, and Information Distribution for Wildfire Detection and Management.” Photogrammatric Engineering & Remote Sensing 64 (10).
Cova, T J. “GIS in Emergency Management,” 18.
Davis, Brett, and Carol Miller. “Modeling Wildfire Probability Using a GIS,” 3.
Gitas, Ioannis Z., Jesús San-Miguel-Ayanz, Emilio Chuvieco, and Andrea Camia. 2014. “Advances in Remote Sensing and GIS Applications in Support of Forest Fire Management.” International Journal of Wildland Fire 23 (5): 603. doi:10.1071/WF14117.
Miller, Carol, and Alan A. Ager. 2013. “A Review of Recent Advances in Risk Analysis for Wildfire Management.” International Journal of Wildland Fire 22 (1): 1. doi:10.1071/WF11114.
Thompson, Matthew P., Jessica R. Haas, Julie W. Gilbertson-Day, Joe H. Scott, Paul Langowski, Elise Bowne, and David E. Calkin. 2015. “Development and Application of a Geospatial Wildfire Exposure and Risk Calculation Tool.” Environmental Modelling & Software 63 (January): 61–72. doi:10.1016/j.envsoft.2014.09.018.
Van Westen, C.J. 2013. “3.10 Remote Sensing and GIS for Natural Hazards Assessment and Disaster Risk Management.” In Treatise on Geomorphology, 259–298. Elsevier. doi:10.1016/B978-0-12-374739-6.00051-8.
Yuan, May. 1997. “Use of Knowledge Acquisition to Build Wildfire Representation in Geographical Information Systems.” International Journal of Geographical Information Science 11 (8): 723–746. doi:10.1080/136588197242059.
Contact
For questions about this annotated bibliography, complied for GEOG560: Introduction to Geographic Information Science, please email Caitlin Barbour