Species Distribution Modeling for Moonworts (Botrychium spp.) an annotated bibliography created for GEOG560
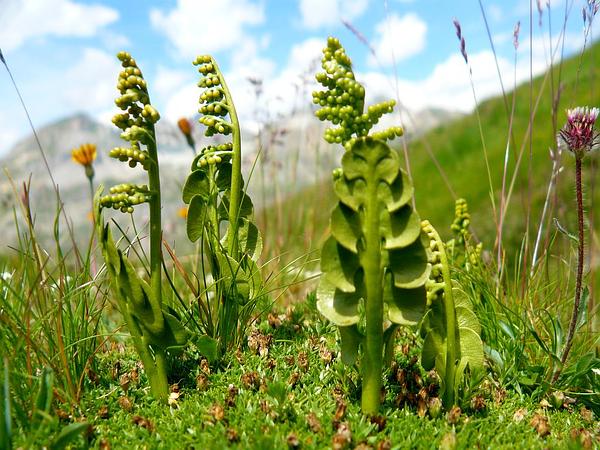
Species Distribution Models (SDM) predict potential suitable habitat for species across a geographical range. A number of geographical and climatic elements (elevation, soils, associated plants, mean temperature, precipitation, etc.) are put into the model and an algorithm is created from the most likely predictors based on known occurrences compared to the wider geographic range. A predictive map is produced indicating areas that share similar suitable habitat. These models help researchers understand what elements may be affecting species presence and help predict future changes to range under climate change. Managers can use SDMs to assess best conservation practices and where to focus search efforts for discovering unknown populations of rare species.
I’m working with the Colville National Forest to develop a Species Distribution Model for moonworts (Botrychium spp.) Moonworts are ephemeral ferns from the Ophioglassaceae family. Although, not all species of moonworts are considered threatened or endangered, many Botrychium species are found colocated and share similar habitat niches. The articles and reports below highlight habits of Botrychium, different species distribution models including their success at finding rare species, and cautionary papers about evaluating SDMs appropriately and choosing elements wisely to avoid collinearity and autocorrelation.
Annotated Bibliography
Ahlenslager, K., Cabral, A., 2012, “Botrychium Monitoring and Information Gaps– Interagency Special Status Species Program Final Report”: Colville National Forest. Unpublished report.
Ahlenslager and Cabral document the population trends, along with effects of climatic conditions and disturbance for five sensitive Botrychium species in the Colville National Forest. Forest employees monitored 12 sites, 6 forested sites and 6 old homestead meadow sites, over the course of two summers. B. crenulatum and B. lineare are found in the western red-cedar/hemlock forests. Thuja plicata in particular is suspected as being an indicator species for moonworts found in the forest. B. hesperium, B. paradoxum, and B. pedunculosum occur at old homestead meadow sites. The moonworts found in meadow sites appear to prefer areas of old disturbance. Plants were not found in areas actively being disturbed by gophers, further monitoring will be needed to see if this type of disturbance will increase future moonwort populations. Associated species were documented at each site: for forested sites Thuja plicata is suspected to be an indicator species for the forest Botrychium while Fragia virginiamum, Fragia vesca, Pinus contorta and Botrychium simplex were frequent in the meadow sites and are also considered potential indicator species. Botrychium tend to be found in clusters of many species, making more common Botrychium an indicator for the rarer species. Previous year’s precipitation did not appear to have an influence on population abundance. Fire ecology for Botrychium was not able to be determined due to a lack of recent fires in the monitored areas. Grazing did not appear to have an effect on meadow populations, overgrazing on the other hand appeared to degrade the soils and negatively impact plants. Other declines in population may be due to shading from forest regeneration, competition with invasive species, an increase in leaf litter depth and less canopy cover from fallen trees in forested sites. This report will be particularly helpful in choosing certain climatic and environmental predictors for a Botrychium SDM. Tables with landform groups, land type associations, and soils for each site are also included.
Bardos, D. C., Guillera-Arroita, G., and Wintle, B. A., 2015, Valid auto-models for spatially autocorrelated occupancy and abundance data: Methods in Ecology and Evolution, v. 6, no. 10, p. 1137-1149. https://dx.doi.org/10.1111/2041-210x.12402
Bardos et al contend with Dormann et al’s argument that auto-logistic regression models and other ‘auto-models’ produce an underestimation of environmental covariation parameters when strong spatial autocorrelation is used. Bardos et al demonstrate that the use of proper neighborhood weighting (e.g. weighted sums instead of weighted means, which were often used in the R package ‘spdep’) consistent with auto-model definitions produce correct model parameters. Similarly, increasing grid density reduces errors due to invalid weighting. Furthermore, they show that Dormann et al’s use of the auto‑Poisson model was inappropriate as it allows only for negative autocorrelation. Bardos et al reviews different auto-models and describes some potential pitfalls that can create errors in relation to spatial autocorrelation and methods to reduce those errors when building a model.
Beale, C. M., and Lennon, J. J., 2012, Incorporating uncertainty in predictive species distribution modelling: Philosophical Transactions of the Royal Society B: Biological Sciences, v. 367, no. 1586, p. 247-258. https://dx.doi.org/10.1098/rstb.2011.0178
Beale et al examine the sources of uncertainty within species distributions models. They define three main methods of creating SDMs: a) Niche-based distribution models which use geographic presence/absence data which use statistical regression to fit predictions of habitat to a mathematical model, b) Demographic models which use population dynamic estimations (e.g. birth, death, fecundity, dispersal rates) to create stochastic patchwork models based on metapopulations and to determine whether a population will thrive or go extinct within a climatic zone, c) Process-based models that describe the physiological components of a species’ niche and build a geographic map based on those components. All these models suffer from uncertainties from: sampling observations, which is often not fully random; covariate parameters; competitive and facilitative interactions with other species; and interpolations of climate data and climate change assumptions. Niche-based models can particularly get into trouble when they misrepresent the importance of covariates in an effort to create a better fit to the mathematical models. The authors suggest that most SDM publications under-estimate uncertainty within the model. The authors recommend that for best management practices clearly stating these uncertainties, while making the model appear less precise avoids overconfidence on a single model’s predictions. I found Beale et al’s paper is very useful in thinking about where potential uncertainties will arise with building an SDM for Botrychium. I will be creating a niche-based model trying to locate extant populations and a realized-niche approach is appropriate for this type of SDM according to this paper.
Bourg, N. A., McShea, W. J., and Gill, D. E., 2005, Putting a Cart before the Search: Successful Habitat Prediction for a Rare Forest Herb: Ecology, v. 86, no. 10, p. 2793-2804, www.jstor.org/stable/3450705
Bourg et al use a classification and regression tree (CART) model to find suitable habitats for a rare orchid in the eastern US. CART models create tree-like diagrams that separate based on likelihood of a certain variable influencing a positive correlation to the presence of your data. Bourg et al used the environmental factors of elevation, aspect, slope, planar curvature of land, soils, forest type, and fire history in their model. They ran multiple simulations to find the tree most suitable based on the minimum cross-validation error rule and the 1 SE rule, which selects the smallest tree within one standard error of the minimum error. The best model created a 13-leaf tree where elevation, slope, forest type, fire frequency and planar convexity most strongly influenced suitable habitat. They evaluated their model by comparing the number of known presence and absence data to the predicted suitable/unsuitable habitats and by ground-truthing, running transects within both predicted suitable and unsuitable areas. The first method showed only a misclassification error of 4.74% and correctly classified 74% of presence and 90% of absence sites. The ground-truthing found 8 new populations within the predicted suitable habitat showing about 20% of the measured suitable habitat to have an orchid population present. Six false negatives were also found, but the authors state those were all near populations in suitable habitat. Bourg et al’s paper presents a possible model (CART) I might use for my own SDM and details the methods for adapting GIS data for the model along with potential areas where CART may not be successful (e.g. smaller populations in small areas.)
Cruz-Cárdenas, G., López-Mata, L., Villaseñor, J. L., and Ortiz, E., 2014, Potential species distribution modeling and the use of principal component analysis as predictor variables: Revista Mexicana de Biodiversidad, v. 85, no. 1, p. 189-199. https://dx.doi.org/10.7550/rmb.36723
Cruz-Cárdenas et al suggest using principal component analysis (PCA) to choose the best model predictors that are not influenced by spatial autocorrelation. Due to the natural spatial autocorrelation, multiple environmental factors may be indicated as being influential predictors within a SDM. Disregarding autocorrelation may: a) increase type I statistical errors, b) incorrectly reject the null hypothesis of no effect, c) select autocorrelated variables may be or give them a higher influence, d) choose coarser scale predictors over more locally influencing predictors, and e) influence the AIC to select models with larger number of variables. PCA orders the principal components (PC) hierarchically according to their variance size. The first PCs selected retain the highest amount of information while reducing the dimensions of the model predictors. Importantly, PCs are independent of each other, removing the autocorrelation effects. The authors then demonstrate how they used PCA to determine seven PCs from an extensive list of predictive environmental variables using a variety of combinations of the environmental and non-spatial factors. The PCs were used to create SDMs using the Maxent algorithim. Since Maxent uses presence-only data they transformed them to Boolean layers (presence-absence) and then validated the model with binomial test. Overall, the models created had precisions above 90% for each species. Cruz-Cárdenas et al paper demonstrates a potentially useful tool, PCA, to help determine the best predictors for creating an SDM while reducing errors from spatial autocorrelation.
Elith, J., Phillips, S. J., Hastie, T., Dudík, M., Chee, Y. E., and Yates, C. J., 2011, A statistical explanation of MaxEnt for ecologists: Diversity and Distributions, v. 17, no. 1, p. 43-57. https://dx.doi.org/10.1111/j.1472-4642.2010.00725.x
MaxEnt’s success as a high performing predictive SDM has made it a popular choice for many researchers. Unlike previous papers that describe MaxEnt through a machine learning lens, this paper explains MaxEnt with statistical terminology for ecologists. MaxEnt uses presence-only data. Presence-only data tends to be more prevalent, particularly with historical records, therefore it is useful to have a SDM that can utilize this data. MaxEnt determines the probability of an environmental variable (aka covariate) (z) where a species is present (f1(z)) over the entire probability density of the covariate (f(z)) within a bounded landscape (L). To determine the probability of presence conditioned on the environment, Pr(y = 1|z), MaxEnt applies transformations, called features, on covariates using six feature classes (linear, product, quadratic, hinge, threshold, and categorical) to best fit a function of species suitability across the landscape. The set of known presence of species data points is L1. MaxEnt automatically picks a background of 10,000 random sample points from L, in which a subsample of L1 is present. MaxEnt uses these background data points to create probability densities for each covariate and estimate the ratio of f1(z)/ f(z), choosing the closest f(z) in order to minimize the relative entropy, which is the same as maximizing the entropy of the raw distribution. MaxEnt incorporates a regularization by applying an error bound to its solutions in order to reduce models that overfit the data. The authors state that the regularization included in MaxEnt makes it less susceptible to errors from autocorrelation. Therefore, extra analysis, like PCA, to preselect covariates is not necessary, although smart selection of variables is recommended. Overall, this paper is useful in describing the theory around MaxEnt and its basic functions. MaxEnt is open-source and can be downloaded here: http://biodiversityinformatics.amnh.org/open_source/maxent/
Fois, M., Fenu, G., Cuena Lombraña, A., Cogoni, D., and Bacchetta, G., 2015, A practical method to speed up the discovery of unknown populations using Species Distribution Models: Journal for nature conservation., v. 24, p. 42-48. https://dx.doi.org/10.1016/j.jnc.2015.02.001
Fois et al used MaxEnt to create an SDM for the rare and threatened plant the yellow gentian in Sardinia. The yellow gentian has been harvested for medicinal purposes and only 8 sites were currently known to exist. The authors chose MaxEnt because it is a generative approach that requires presence-only data and it has demonstrated being a robust method for other species with small sample sizes. Environmental variables were selected with Variance Inflation Factor (VIF) and Percent of Contribution indexes were used to reduce overfitting of the model. Due to the small sample size they evaluated their model with the ‘leave-one-out’ n-fold test. They used their initial model to then investigate the predicted sites for yellow gentian. After locating 16 new occurrences and considering historical records that fell within the model predictions as extinct, the authors ran 2 more model simulations with the expanded data sets. All three returned high area under the curve (AUC)s values. The best observed post predictive power (the true positive sites/total predicted positive sites) occurred in the largest data set that included the historical sites. The authors stated that using MaxEnt was a useful tool to more quickly identify new sites of rare species, saving time and money, but is not a replacement for expertise. This paper suggests that MaxEnt is likely a good SDM to use for rare Botrychium species, where known sites are limited. The observed post predictive test is an interesting method to evaluate the model, but can lead to false high performance rates if the grid resolution is too coarse.
Gogol-Prokurat, M., 2011, Predicting habitat suitability for rare plants at local spatial scales using a species distribution model: Ecological Applications, v. 21, no. 1, p. 33-47. https://dx.doi.org/10.1890/09-1190.1
The Pine Hill Preserve is a conservation area within the Pine Hill Complex (PHC), which is composed of gabbroic soils that support edaphic endemic rare plant species. In order to expand the preserve and maximize protection of endemic species, the managers need accurate information to best direct the acquisition of surrounding private lands. Since plant surveys are not allowed on private property, M. Gogol-Prokurat developed varying SDMs for 4 of the edaphic PHC species. She compares various MaxEnt models’ accuracy in determining and ranking habitat suitability for each species based on extent, regional vs. local, and resolution of environmental variables, coarse (823m) vs. fine-grain (30m), simulated across three model types (climate-only, soils-only, and full models). She then reviews their usefulness for conservation efforts examining each model’s AUC and testing for goodness of fit from Hosmer-Lemeshow deciles using a Fisher’s Exact test of independence. The author points out that the AUC’s often reflect a spatial bias due to the fact that test pseudo-absences are randomly distributed across the entire extent while the test presences are spatially clustered. To correct for this bias, the author calculated a second AUC using independent presence/absence data within the PHC. She found that the independent AUC showed more accurate discrimination of models than the typical AUC method. The soils-only model gave good results for 3 out of the 4 PHC species. Adding the fine-grain predictors improved models for 2 of the 4 species and the climate-only models also performed well. The fine-grain, full models had better discrimination than the coarse-grain models for 3 of the 4 species and were more precise. Larger spatial extent increased the goodness of fit, even with species that have very localized populations, while fine-grain environmental variables improved local-scale discrimination. Gogol-Prokurat suggests that using multiple model designs and over-laying their results can help direct where to best apply conservation efforts. She, also, cautions that for species that have disturbance driven dispersal and persist temporally through seed banks aren’t as easily modeled in SDMs. This last note reflects Botrychiums, in that they tend to persist as sporophytes in the soil and do not produce vegetative traits every year.
Hernandez, P. A., Graham, C. H., Master, L. L., and Albert, D. L., 2006, The effect of sample size and species characteristics on performance of different species distribution modeling methods: Ecography, v. 29, no. 5, p. 773-785. https://dx.doi.org/10.1111/j.0906-7590.2006.04700.x
The method used to model species distribution can have significant effects on the predictive map created. For rare species in particular, researchers often have to contend with small sample size and the availability of presence-only data, which affect the robustness of the model. Hernandez et al tested 4 model methods (Bioclim, Domain, GARP, and MaxEnt) using varying sample sizes (5, 10, 25, 50, 75, 100) on 18 rare California animal species to determine how well each model performed based on sample size. The models were evaluated three ways: 1) calculating the AUC of the receiver operating characteristic (ROC) plots–ROC plots compare the sensitivity (true-positives) versus the specificity (false-positives); 2) the predictive success, which is the percent of positive evaluation occurrences correctly classified as positive; 3) and spatially comparing each model’s binary suitability maps to a “full” model including 150 occurrences. The authors also examined the effects of marginality, tolerance, and spatial extent of each taxa on the SDMs. Overall, MaxEnt outperformed all the other models by creating relatively stable predictions across all samples sizes and most accurately predicted presence when compared to the full model, even with a sample size of 5. The predicted area of occurrence increased the least as sample size increased compared to the other models. Domain, also, performed well in regards to the AUC evaluations and tended to create the smaller predicted areas compared to MaxEnt. Models for species that were more marginal and had lower tolerances and smaller spatial extents tended to perform better than those with larger extents and less marginal. These results suggest that SDMs are particularly robust tools for predicting habitat for rare, specialist species compared to generalist. The results of Hernandez et al’s paper reinforces that MaxEnt is one of the best methods to use for creating an SDM for Botrychium.
Jarnevich, C. S., Stohlgren, T. J., Kumar, S., Morisette, J. T., and Holcombe, T. R., 2015, Caveats for correlative species distribution modeling: Ecological Informatics, v. 29, p. 6-15. https://dx.doi.org/10.1016/j.ecoinf.2015.06.007
This paper highlights four main caveats that researchers should keep in mind when building and analyzing SDMs. First, that “all sampling data are incomplete and potentially biased.” Ideally, sampling should capture both the geographical range and the environmental gradients where the species are found, but sampling constraints of time, money, and accessibility can limit capturing a full data set. Absence data may not represent true absence due to temporal, life cycle, or misidentification; but the authors conclude it is still better to include possibly faulty absence-data when available. Second, “predictor variables must capture distribution constraints.” Collinearity and spatial autocorrelation create problems for models which assume independence amongst variables. Third, “no single model works best for all species in all areas at all spatial scales and over time.” Models assume equilibrium conditions and equitable sampling across environmental gradients but these assumptions are often difficult to meet. Therefore, when possible, sampling bias should be mimicked in the selection of background or pseudo-absence sites. For best results, researches should compare models using multiple modeling techniques and evaluation statistics. Finally, “the results of species distribution models should be treated like a hypothesis to be tested and validated with additional sampling and modeling in an iterative process.” Essentially, SDMs show correlations between environmental factors and presence of a species. Researchers should not use these as final conclusions but leads to explore these potential relationships. Recognizing where and how errors enter into models helps us to better understand the extent of usefulness of these models for making conservation decisions and can direct how to accommodate for limitations and inform further research.
Jiménez-Valverde, A., Lobo, J. M., and Hortal, J., 2008, Not as Good as They Seem: The Importance of Concepts in Species Distribution Modelling: Diversity and Distributions, v. 14, no. 6, p. 885-890, www.jstor.org/stable/20172048
In this paper, Jimenez-Valverde et al question the conclusions of Tsoar et al (2007) that complex techniques create better predictive distribution maps than simple methods and that the predictive distribution maps are more accurate for habitat specialist than generalist species. The authors contend that the evaluation techniques used to determine model accuracy (e.g. AUC, kappa statistic), by nature of subjective thresholds, will favor more complex algorithms due to overfitting of the model. Similarly, these validation techniques inherently favor models for specialist species because of the number of occurrences relative to absences across the extent are greater. Furthermore, simply increasing the extent of the study area improves the validation score. They suggest evaluating models based on errors of omission and commission separately and accounting for the relative occurrence area, the ratio between the extent of occurrence and the entire extent of the study area. Jimenez-Valverde et al encourages modelers to reflect on the validation process of SDMs so that we don’t fall into false assumptions of accuracy.
Johnson-Groh, C., Riedel, C., Schoessler, L., and Skogen, K., 2002, Belowground Distribution and Abundance of Botrychium Gametophytes and Juvenile Sporophytes: American Fern Journal, v. 92, no. 2, p. 80-92, 13, https://doi.org/10.1640/0002-8444(2002)092[0080:BDAAOB]2.0.CO;2
Understanding the population dynamics of Botrychium spp. can lead to important clues to selecting environmental variables for an SDM. Johnson-Groh et al’s paper explains two important aspects of moonworts. First, they have an extensive belowground distribution compared to their aboveground vegetative structures. Botrychium spores, sporophytes, asexual gemmae, and even juvenile plants spend years growing belowground before sending up green leaves. Secondly, the authors conclude based on their belowground habits, that Botrychium spp. are highly dependent on mycorrhizae for nutrients and growth. Understanding the type of mycorrhizae Botrychium utilize and then incorporating the distribution of those mycorrhizae into an SDM would create a more robust predictive map, but this data may be unavailable and hard to attain. A few other points from this paper I would like to highlight are: a) the authors don’t think aboveground disturbances such as fire and herbivory greatly impact Botrychium populations; b) gametophytes can self-fertilize, so a single spore can potentially start a new colony; c) certain species rely heavily on asexual reproduction, which leads to a trade-off of short-term exploitation of a suitable habitat versus long-term evolution from dispersal of spores.
McCune, J. L., 2016, Species distribution models predict rare species occurrences despite significant effects of landscape context: Journal of Applied Ecology, v. 53, no. 6, p. 1871-1879. https://dx.doi.org/10.1111/1365-2664.12702
In this paper, J.L. McCune explored the effectiveness of SDMs to detect suitable habitat for rare forest plants based on climatic and soil data compared to plant community associations and habitat fragmentation. For each of the 8 rare species, she built seven slightly varied models using MaxEnt using 14 minimally autocorrelated predictors at a 100 m2 resolution. Each of the 10 replicate runs excluded a random 25% of the presence data, in order to test the accuracy of the run. She used the cumulative model output in order to avoid assumptions about species prevalence. After modelling, 51 grid squares of predicted suitable habitat were randomly selected and thoroughly searched for presence of rare species. All vascular plant species were also documented and ranked according by abundance to estimate to the plant community. A habitat was considered fragmented if a grid square had less than 40 ha of forest cover within 500 m. Fragmentation was calculated using ArcGIS. McCune then built a generalized linear model (GLM) based on independent presence-absence data to compare the results of the MaxEnt models to associated plants and habitat fragmentation. Overall, for 7 of the 8 rare species MaxEnt was successful in determining suitable habitat from climatic and soils data only. The associated plants only had positive correlations for 5 of the 8 species and habitat fragmentation was an important predictor for 2 species. Distance to nearest observed occurrence also had a significant effect on presence within suitable habitat for most of the species, suggesting dispersal may be an important factor. For the one species where the model did not perform well, McCune hypothesizes that based on declining occurrences the species is in the process of becoming extirpated from the landscape. I emphasized the methods of this paper because I expect to use similar methods when building a model for Botrychium. McCune did an excellent job explaining her methods clearly and concisely. I, also, find it interesting that the associated plant communities did not provide a stronger indicator for suitability due to a lot of community variation between sites and am curious to see if moonworts show a strong correlation to particular plants.
Merow, C., Smith, M. J., and Silander, J. A., 2013, A practical guide to MaxEnt for modeling species’ distributions: what it does, and why inputs and settings matter: Ecography, v. 36, no. 10, p. 1058-1069. https://dx.doi.org/10.1111/j.1600-0587.2013.07872.x
Many researchers tend to choose the default settings when using the MaxEnt program. Merow et al advise choosing parameters to best suit the species and type of question one is looking to answer. The authors review how MaxEnt works and how to choose the following model settings. a) Background sample should reflect the environmental conditions one is interested comparing to species presence. b) One should minimize correlated variables prior to building the model. Also, choose simple linear or quadratic transformation features to reduce overfitting. c) A number of regularization values should be explored to maximize fit based on the cross-validation data set. d) Account for sampling bias using methods based on Target Group Sampling or from direct sample measures. e) Use the MaxEnt raw output of Relative Occurrence Rate (ROR) whenever possible and avoid the logistic output because of the post-processing assumptions. f) Evaluate the models predictive accuracy on statistically independent data sets, or use the K-fold cross validation method. Avoid setting thresholds and be wary of AUC unless the species is near range equilibrium and has been sampled intensively. This paper provides insightful guidance on building a spatial model in MaxEnt so that the best predictions can be made based on the type of question being asked.
Naimi, B., and Araújo, M. B., 2016, sdm: a reproducible and extensible R platform for species distribution modelling: Ecography, v. 39, no. 4, p. 368-375. https://dx.doi.org/10.1111/ecog.01881
Naimi and Araújo have built sdm, an in depth R program that allows users to create and modify different SDMs with a single package. sdm appears to be a powerful tool in that it allows researchers to readily compare models created by various methods easily and within a single program. Users can choose from 15 modelling methods including GLM, MaxEnt, Bioclim, Domain, CART, Ensemble, community-based models to name a few. To allow for more accurate comparison of models, sdm has made the feature construction (e.g. linear, quadratic, polynomial, hinge) independent from the specific models. It also includes pre-processing features to detect collinearity, create pseudo-absences, and identify and correct potential errors from differing coordinate systems and spatial extents. In addition, several post-processing to analyze the models are included in sdm, such as sensitivity, specificity, AUC, and calculating p-values with Jackknife. For ease of use, sdm has a GUI interface for researchers who are not as familiar with coding in R. Overall, sdm sounds like an excellent one-stop package for spatial modelers. I like that it is based in R, which is a popular programming language amongst environmental scientists. I’m curious to see how well sdm has worked for other researchers and will be looking for papers that mention using the sdm software package.
Sousa-Silva, R., Alves, P., Honrado, J., and Lomba, A., 2014, Improving the assessment and reporting on rare and endangered species through species distribution models: Global Ecology and Conservation, v. 2, p. 226-237. https://dx.doi.org/https://doi.org/10.1016/j.gecco.2014.09.011
The European Union has a Habitats Directive that requires each member state to maintain and restore natural habitats and conserve rare species and report on their progress. Past reporting standards used a minimum convex polygon (MCP) to map species ranges, which often resulted in gross overestimates particularly for rare species with patchy distributions. Currently the EU uses Range Tool which has standardized the reporting of occurrences and creates more realistic range maps for species. Both Range Tool and MCP do not take in account climate change scenarios that are likely to affect the range of rare and endangered species in the future. Sousa-Silva et al used SDM to model the suitable habitat of a rare plant located in Portugal’s Iberian Peninsula to determine the value of SDMs in identifying the species range from 2000 and the potential change in range for 2020 and 2050. The authors created three models: a partial regional scale model based on climatic data, a partial local scale model based on landscape predictors, and a combined model created by the overlap of the two models. They used an Ensemble method using the BIOMOD package in R to combine 10 different modelling methods (GLM, GAM, CTA, ANN, SRE, GBM, RF, MARS, MDA and MAXENT) into a single predictive output. The SDM was effective in predicting the current known occurrences for 2000 and predicted a 21% in loss of habitat by 2050. The current predicted area was greater than that created by Range Tool, but the authors suggest that there may be unknown populations within that suitable habitat. They conclude that SDMs are useful tool for land managers to make informed conservation decisions, understand potential threats to species due to climate change and to discover unknown populations. Two of the most interesting aspects of this paper were 1) that the authors used a two different scales to model climatic predictors and landscape predictors and 2) they combined 10 different model methods to create a final model based on the idea that combining methods creates a more robust spatial model.
Winther, J. L., and Friedman, W. E., 2007, Arbuscular mycorrhizal symbionts in Botrychium (Ophioglossaceae): American Journal of Botany, v. 94, no. 7, p. 1248-1255. https://dx.doi.org/10.3732/ajb.94.7.1248
Winther and Friendman identified symbiont arbuscular mycorrhizae (AM) in two moonwort species, B. crenulatum and B. lanceolatum. DNA sequencing showed 11 different phylotypes within a single clade of Glomus associated with the Botrychium species. Interestingly, 10 of the Glomus AM phylotypes are associated with Arachnitis uniflora, a mycoheterotrophic angiosperm native to the Falkland Islands, suggesting that these Glomus phylotypes specialize in associating with non-photosynthetic species. The authors also found a few of the Glomus phylotypes in the photosynthetic Calthas palustris plants nearby the Botrychium species. The authors hypothesized that the Botrychium plants use Glomus AM as a way of getting nutrients from photosynthetic plants nearby, but indicated more research is needed to determine the carbon flow. This paper demonstrates that there is still a we don’t fully understand in regards to the symbiotic relations Botrychium has with arbuscular mycorrhizae and their greater plant community. An SDM could potentially point out with which plants Botrychium have a greater association and test them for shared mycorrhizae.
Additional Resources
Beauvais, G. P., Keinath, D. A., Hernandez, P., Master, L., and Thurston, R. 2004. ELEMENT DISTRIBUTION MODELING: A PRIMER. Unpublished manuscript. https://transfer.natureserve.org/download/quarterly/EDM_workshops/edm_primer_v1 _040907.pdf
de Siqueira, M. F., Durigan, G., de Marco Júnior, P., and Peterson, A. T., 2009, Something from nothing: Using landscape similarity and ecological niche modeling to find rare plant species: Journal for Nature Conservation, v. 17, no. 1, p. 25-32. https://dx.doi.org/10.1016/j.jnc.2008.11.001
Felicisimo, A., and Gómez-Muñoz, A., 2004, GIS and predictive modelling: a comparison of methods applied to forestal management and decision-making, Conference Paper. https://www.researchgate.net/publication/232423523
Lescia, P., 1995, “Monitoring Botrychium crenulatum and B. pedunculosum on Colville National Forest”: Colville National Forest. Unpublished report.
Madon, B., Warton, D. I., and Araújo, M. B., 2013, Community-level vs species-specific approaches to model selection: Ecography, v. 36, no. 12, p. 1291-1298. https://dx.doi.org/10.1111/j.1600-0587.2013.00127.x
Pecchi, M., Marchi, M., Burton, V., Giannetti, F., Moriondo, M., Bernetti, I., Bindi, M., and Chirici, G., 2019, Species distribution modelling to support forest management. A literature review: Ecological Modelling, v. 411, p. 108817. https://dx.doi.org/10.1016/j.ecolmodel.2019.108817
Whittier, P., 1984, The Organic Nutrition of Botrychium Gametophytes: American Fern Journal, v. 74, no. 3, p. 77-86. https://dx.doi.org/10.2307/1546541
Zimmermann, N. E., Edwards, T. C., Graham, C. H., Pearman, P. B., and Svenning, J.-C., 2010, New trends in species distribution modelling: Ecography, v. 33, no. 6, p. 985-989, http://www.jstor.org/stable/25790080